Large-scale commodity identification method based on deep learning
A technology of deep learning and recognition methods, applied in the field of image recognition, can solve the problems of high equipment requirements, slow operation speed, large workload, etc., to achieve the effect of reducing training difficulty, stable recognition ability, and improving model accuracy
- Summary
- Abstract
- Description
- Claims
- Application Information
AI Technical Summary
Problems solved by technology
Method used
Image
Examples
Embodiment 1
[0051] Such as figure 1 As shown, a large-scale product recognition method based on deep learning includes the following steps:
[0052] S1: Establish a product detection model, the specific method includes the following steps:
[0053] S1-1: Perform data enhancement processing on the existing multi-scenario and multi-commodity dataset, which includes pictures of various commodities under different backgrounds and lighting environments;
[0054] Divide the multi-scenario and multi-commodity data set into a detection network training set and a detection network test set. The detection network training set includes no less than 90,000 training pictures, and the training pictures include no less than 1,300 types of goods and scene data;
PUM
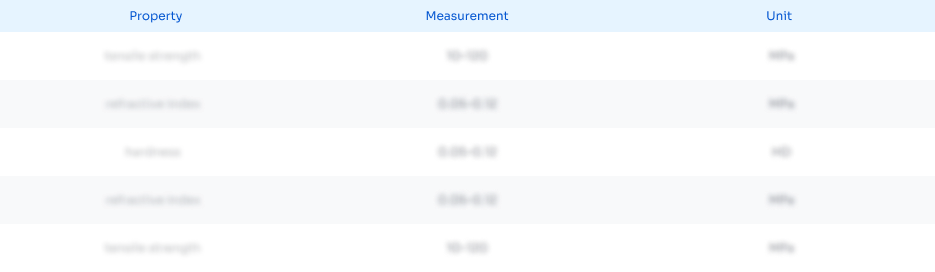
Abstract
Description
Claims
Application Information
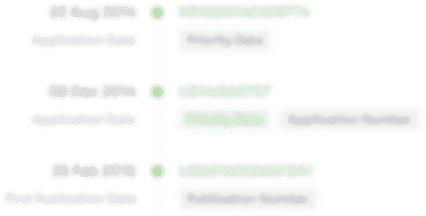
- R&D Engineer
- R&D Manager
- IP Professional
- Industry Leading Data Capabilities
- Powerful AI technology
- Patent DNA Extraction
Browse by: Latest US Patents, China's latest patents, Technical Efficacy Thesaurus, Application Domain, Technology Topic, Popular Technical Reports.
© 2024 PatSnap. All rights reserved.Legal|Privacy policy|Modern Slavery Act Transparency Statement|Sitemap|About US| Contact US: help@patsnap.com