Intelligent Fault Diagnosis Method Based on Attention Mechanism Meta-learning Model in Small Samples
An attention mechanism and intelligent diagnosis technology, applied in biological neural network models, computer parts, character and pattern recognition, etc., can solve problems such as low generalization of algorithms, small number of samples, and complex working conditions.
- Summary
- Abstract
- Description
- Claims
- Application Information
AI Technical Summary
Problems solved by technology
Method used
Image
Examples
Embodiment
[0068] Take a motor bearing fault data set as an example to illustrate. This data set contains the data of three bearing operating states: normal, inner ring fault, and outer ring fault. At the same time, the mechanical signals of the corresponding operating states are collected at three speeds (10Hz, 20Hz, 30Hz). The running state contains 155 samples, and a total of 1395 samples. Take 15 samples at 30Hz speed as training data, and the remaining 450 samples as test data to construct a data set at the same speed; take 45 samples mixed with three speeds as training data, and the remaining 1350 samples as test The data is constructed as a data set at mixed speeds. The training sample data volume only accounts for 3.2% of the total sample data volume.
[0069] Such as figure 1 Shown, the present invention comprises the following steps:
[0070] Step 1: First, perform short-time Fourier transform on the acquired data set to obtain its time-frequency diagram, then use the Resiz...
PUM
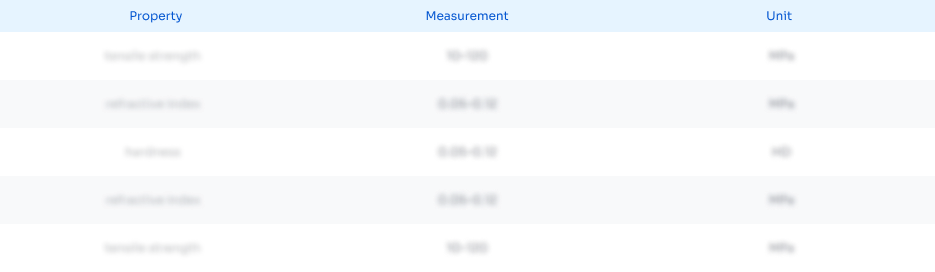
Abstract
Description
Claims
Application Information
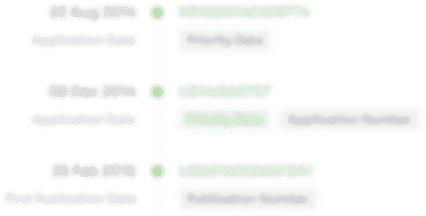
- R&D Engineer
- R&D Manager
- IP Professional
- Industry Leading Data Capabilities
- Powerful AI technology
- Patent DNA Extraction
Browse by: Latest US Patents, China's latest patents, Technical Efficacy Thesaurus, Application Domain, Technology Topic, Popular Technical Reports.
© 2024 PatSnap. All rights reserved.Legal|Privacy policy|Modern Slavery Act Transparency Statement|Sitemap|About US| Contact US: help@patsnap.com