End-to-end weak supervision target detection method based on frame regression of deep learning
A technology of deep learning and target detection, applied in neural learning methods, instruments, biological neural network models, etc., can solve problems such as reducing target detection efficiency and increasing target detection time
- Summary
- Abstract
- Description
- Claims
- Application Information
AI Technical Summary
Problems solved by technology
Method used
Image
Examples
Embodiment Construction
[0032] The present invention will be further described in detail below in conjunction with the accompanying drawings and embodiments.
[0033] The present invention proposes an end-to-end weakly supervised target detection method based on frame regression based on deep learning. By introducing a pseudo-labeled frame as the supervision condition of the weakly supervised target detection network, the frame regression network structure is introduced, thereby combining the two steps The weakly supervised detection model is simplified to an end-to-end network model structure, which not only simplifies the network model. The time for weakly supervised target detection is reduced, and the detection accuracy of the model is increased by entering the border regression network and the border regression loss function. Improve the efficiency of weakly supervised object detection network. The flow chart of the implementation steps of the weakly supervised target detection network is as fo...
PUM
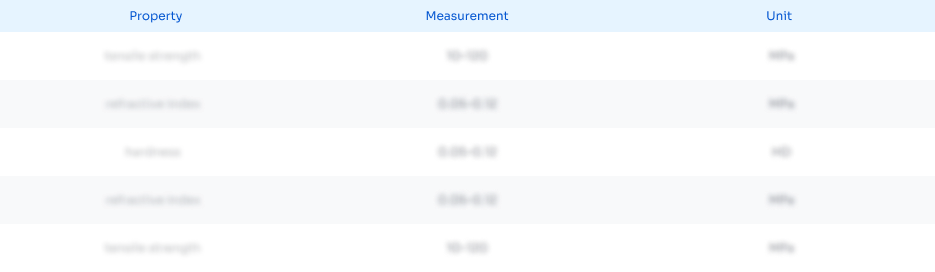
Abstract
Description
Claims
Application Information
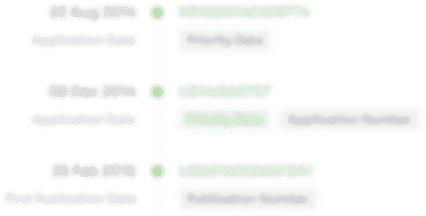
- R&D Engineer
- R&D Manager
- IP Professional
- Industry Leading Data Capabilities
- Powerful AI technology
- Patent DNA Extraction
Browse by: Latest US Patents, China's latest patents, Technical Efficacy Thesaurus, Application Domain, Technology Topic, Popular Technical Reports.
© 2024 PatSnap. All rights reserved.Legal|Privacy policy|Modern Slavery Act Transparency Statement|Sitemap|About US| Contact US: help@patsnap.com