Atmospheric pollutant concentration prediction method integrating machine learning with LSTM
A technology of air pollutants and machine learning, applied in machine learning, neural learning methods, forecasting, etc., can solve the problem of less spatiotemporal feature mining of data distribution, achieve fast calculation speed, simple data, and improve accuracy
- Summary
- Abstract
- Description
- Claims
- Application Information
AI Technical Summary
Problems solved by technology
Method used
Image
Examples
Embodiment Construction
[0054] It should be noted that, in the case of no conflict, the embodiments of the present invention and the features in the embodiments can be combined with each other.
[0055] The present invention will be described in detail below with reference to the accompanying drawings and examples.
[0056] The present invention firstly defines the air pollutant concentration prediction.
[0057]Concentration prediction of air pollutants: By learning the relationship between historical pollutant monitoring data, the concentration of air pollutants such as PM2.5, PM10, and SO2 can be predicted within a certain period of time in the future.
[0058] Traditional prediction methods: The predictions based on the physical diffusion model and chemical reaction of pollutants are collectively referred to as traditional prediction methods.
[0059] A prediction method of atmospheric pollutant SO2 concentration that integrates machine learning and LSTM, such as Figure 1-3 as shown,
[0060]...
PUM
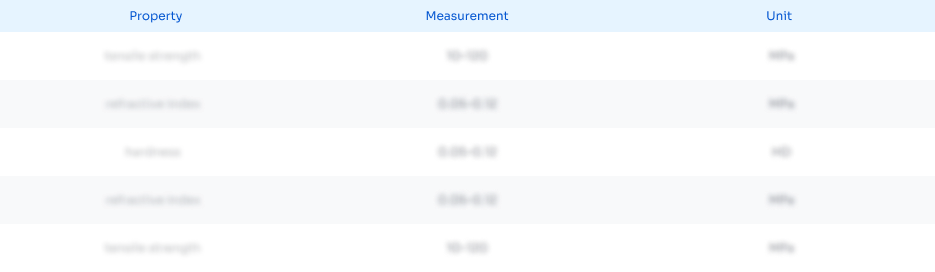
Abstract
Description
Claims
Application Information
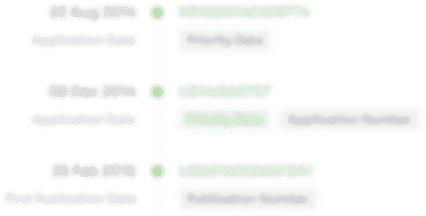
- R&D Engineer
- R&D Manager
- IP Professional
- Industry Leading Data Capabilities
- Powerful AI technology
- Patent DNA Extraction
Browse by: Latest US Patents, China's latest patents, Technical Efficacy Thesaurus, Application Domain, Technology Topic, Popular Technical Reports.
© 2024 PatSnap. All rights reserved.Legal|Privacy policy|Modern Slavery Act Transparency Statement|Sitemap|About US| Contact US: help@patsnap.com