DCF tracking confidence evaluation and classifier updating method based on neural network
A neural network and update method technology, applied in neural learning methods, biological neural network models, instruments, etc., can solve the problems of lack of tracking confidence evaluation measures, low efficiency, and large interference.
- Summary
- Abstract
- Description
- Claims
- Application Information
AI Technical Summary
Problems solved by technology
Method used
Image
Examples
Embodiment
[0064] A neural network-based method for DCF tracking confidence evaluation and classifier update. First, a convolutional neural network for response graph analysis for evaluating tracking confidence is designed and trained. After the network training is completed, it is used to evaluate and adaptively update the tracking confidence of the correlation filter.
[0065] First, the training data set is established based on the response map generated by the common correlation filtering method. When the distance between the estimated position and the actual center position of the target is less than 1 / 5 of the diagonal length of the target, and the shape is close to the ideal two-dimensional Gaussian function response The map is used as a positive class; the distance between the estimated position and the actual center position of the target is greater than 1 / 3 of the diagonal length of the target, and the response map with a very rough and irregular shape is used as a positive clas...
PUM
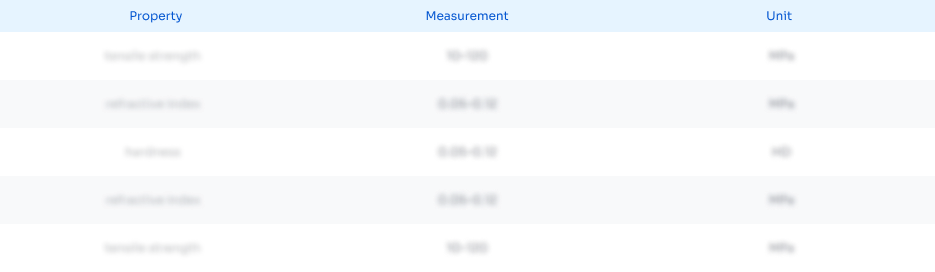
Abstract
Description
Claims
Application Information
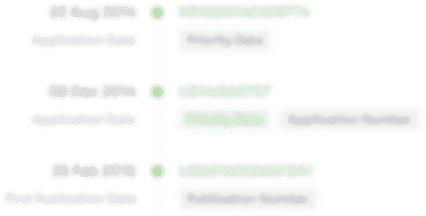
- R&D Engineer
- R&D Manager
- IP Professional
- Industry Leading Data Capabilities
- Powerful AI technology
- Patent DNA Extraction
Browse by: Latest US Patents, China's latest patents, Technical Efficacy Thesaurus, Application Domain, Technology Topic.
© 2024 PatSnap. All rights reserved.Legal|Privacy policy|Modern Slavery Act Transparency Statement|Sitemap