Medical image segmentation method based on improved convolutional neural network
A convolutional neural network and medical image technology, applied in image analysis, image enhancement, image data processing, etc., can solve problems such as deepening and gradient disappearance
- Summary
- Abstract
- Description
- Claims
- Application Information
AI Technical Summary
Problems solved by technology
Method used
Image
Examples
Embodiment Construction
[0040] The specific implementation of the present invention will be described in detail below in conjunction with the accompanying drawings.
[0041] Such as figure 1 As shown, the present invention discloses a convolutional neural network based on deep learning and applied to the segmentation task of medical images. The construction method of the convolutional neural network includes: Step A to build the overall network architecture; Step B to build the framework of the multi-channel fusion module; Step C to build the framework of densely connected multi-channel fusion modules; Step D to build the down-sampling module and up-sampling module framework; Step E builds each module into the whole network framework. The training and testing steps of the convolutional neural network include: step F initializes and preprocesses the training set; step G inputs the training set into the network, and adjusts the hyperparameters of the network so that the network can obtain the best con...
PUM
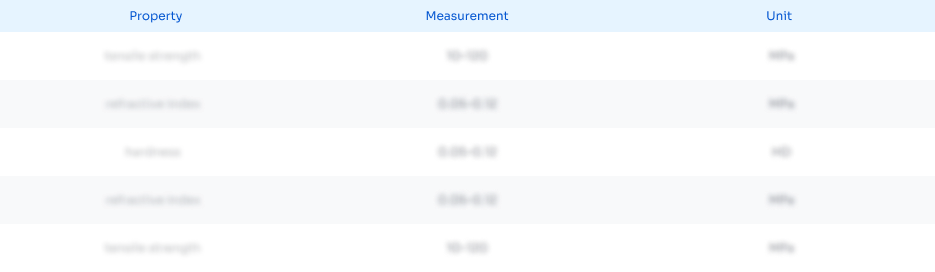
Abstract
Description
Claims
Application Information
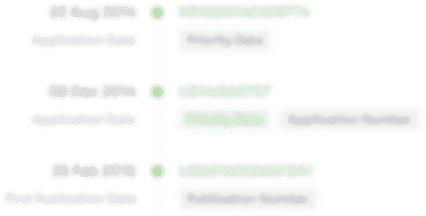
- R&D Engineer
- R&D Manager
- IP Professional
- Industry Leading Data Capabilities
- Powerful AI technology
- Patent DNA Extraction
Browse by: Latest US Patents, China's latest patents, Technical Efficacy Thesaurus, Application Domain, Technology Topic.
© 2024 PatSnap. All rights reserved.Legal|Privacy policy|Modern Slavery Act Transparency Statement|Sitemap