CNN model, training method thereof, terminal and computer readable storage medium
A training method and model technology, applied in the field of CNN model training, can solve the problems of reduced model training performance and high economic cost, and achieve the effect of ensuring the effect of batch normalization
- Summary
- Abstract
- Description
- Claims
- Application Information
AI Technical Summary
Problems solved by technology
Method used
Image
Examples
Embodiment 1
[0051] A CNN model training method of the present invention is applied to a CNN model with a linear structure, and the CNN model has a batch normalization layer.
[0052] The training method performs model segmentation and data batching on the CNN model according to the size of batch training samples and computer storage resources.
[0053] Among them, the model segmentation is: a synchronization layer is inserted between each batch normalization layer and the previous network layer adjacent to it, and the synchronization layer is used to temporarily store the output of the previous network layer adjacent to it, and is used for Start the batch operation of the input data by the batch normalization layer adjacent to it; all network layers between the input layer and its adjacent synchronization layer, and all network layers between any two adjacent synchronization layers are respectively A single network layer unit.
[0054] Data batching is: For all the above network layer un...
Embodiment 2
[0085] In the second aspect, a CNN model of the present invention has a linear structure, and is a model obtained through training of a CNN model training method disclosed in Embodiment 1.
[0086] In this embodiment, the CNN model includes an input layer, a convolutional layer, a fully connected layer, an activation layer, a batch normalization layer and a synchronization layer.
[0087] The input layer is used to input training samples, the convolutional layer and the fully connected layer are used to perform convolution calculations to extract features, and the total number of convolutional layers and fully connected layers is N; the order of the activation layer and the convolutional layer and the fully connected layer Stacking; there are a total of N batch normalization layers, and each batch normalization layer is located behind its corresponding convolutional layer and fully connected layer without intervals; there are N synchronization layers and one-to-one corresponden...
Embodiment 3
[0099] A terminal of the present invention includes a processor, an input device, an output device and a memory, the processor, the input device, the output device and the memory are connected to each other, the memory is used to store a computer program, the computer program includes program instructions, and the processor is configured to The method for training a CNN model as disclosed in Embodiment 1 is executed by calling the above-mentioned program instructions.
PUM
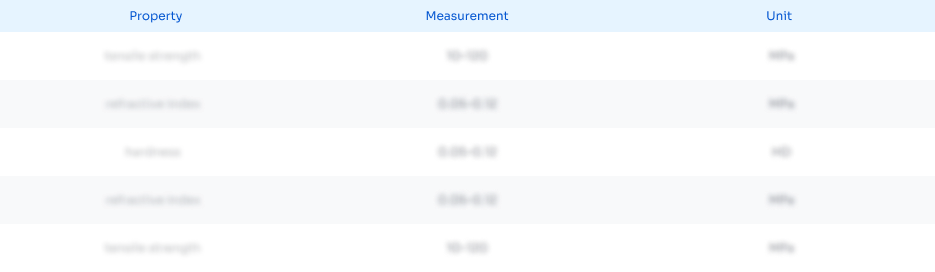
Abstract
Description
Claims
Application Information
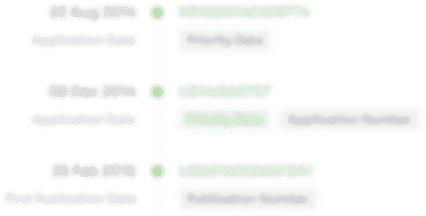
- R&D
- Intellectual Property
- Life Sciences
- Materials
- Tech Scout
- Unparalleled Data Quality
- Higher Quality Content
- 60% Fewer Hallucinations
Browse by: Latest US Patents, China's latest patents, Technical Efficacy Thesaurus, Application Domain, Technology Topic, Popular Technical Reports.
© 2025 PatSnap. All rights reserved.Legal|Privacy policy|Modern Slavery Act Transparency Statement|Sitemap|About US| Contact US: help@patsnap.com