Steam turbine health state prediction method based on E-LSTM
A prediction method and health status technology, applied in prediction, biological neural network models, data processing applications, etc., can solve problems such as slow system operation, opaque rule relations, and difficulty in fully exploring internal connections
- Summary
- Abstract
- Description
- Claims
- Application Information
AI Technical Summary
Problems solved by technology
Method used
Image
Examples
Embodiment Construction
[0039] The following examples describe the present invention in more detail.
[0040] The present invention is an E-LSTM-based steam turbine health degree prediction method, and its structure diagram is as follows figure 1 shown, including collecting steam turbine operating data and obtaining the distribution characteristics of its health status;
[0041] In order to overcome the defects existing in the prior art, the present invention proposes a steam turbine health state prediction model E-LSTM on the basis of predecessors' research, that is, by using a long-short-term memory neural network (LSTM) training model combined with an evolutionary algorithm ( Evolutionary algorithms) model selection, to achieve the purpose of improving the prediction accuracy and reducing over-fitting, the present invention adopts the following steps to realize the state prediction of the steam turbine:
[0042] Step 01. Collect turbine operation data from sensors and preprocess the data.
[004...
PUM
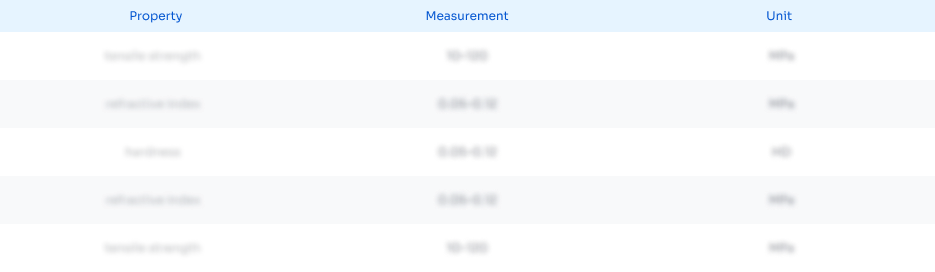
Abstract
Description
Claims
Application Information
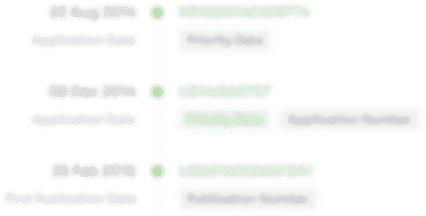
- R&D
- Intellectual Property
- Life Sciences
- Materials
- Tech Scout
- Unparalleled Data Quality
- Higher Quality Content
- 60% Fewer Hallucinations
Browse by: Latest US Patents, China's latest patents, Technical Efficacy Thesaurus, Application Domain, Technology Topic, Popular Technical Reports.
© 2025 PatSnap. All rights reserved.Legal|Privacy policy|Modern Slavery Act Transparency Statement|Sitemap|About US| Contact US: help@patsnap.com