A fault diagnosis method based on semi-supervised learning deep adversarial network
A semi-supervised learning and fault diagnosis technology, applied in neural learning methods, biological neural network models, testing of mechanical components, etc., can solve problems such as unrealistic collection and unlabeled vibration data
- Summary
- Abstract
- Description
- Claims
- Application Information
AI Technical Summary
Problems solved by technology
Method used
Image
Examples
Embodiment Construction
[0068] The technical solutions in the embodiments of the present invention will be clearly and completely described below with reference to the accompanying drawings in the embodiments of the present invention. Obviously, the described embodiments are only a part of the embodiments of the present invention, rather than all the embodiments. Based on the embodiments of the present invention, all other embodiments obtained by those of ordinary skill in the art without making creative efforts shall fall within the protection scope of the present invention.
[0069] Depend on figure 1 and figure 2 As shown, a fault diagnosis method based on a semi-supervised learning deep adversarial network of the present invention includes the following specific steps:
[0070] S1, obtain the total set of samples containing k types of bearing faults Y={Y 1 ,Y 2 ,Y 3 ,…Y k}, that is, Y={Y i}, i=1, 2, 3,...k; in this embodiment, the bearing fault category k=50;
[0071] Y i represents the ...
PUM
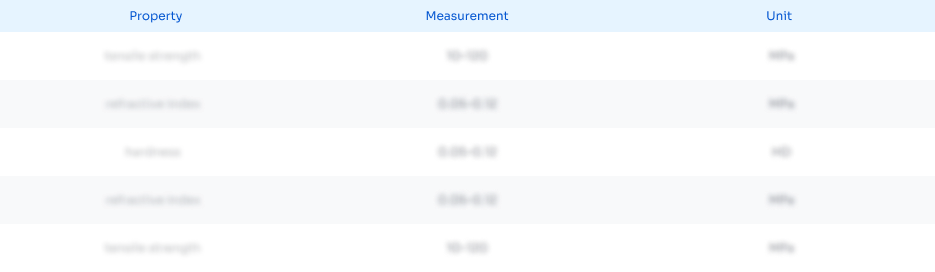
Abstract
Description
Claims
Application Information
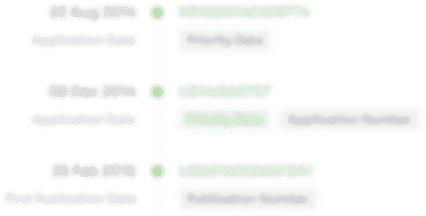
- R&D Engineer
- R&D Manager
- IP Professional
- Industry Leading Data Capabilities
- Powerful AI technology
- Patent DNA Extraction
Browse by: Latest US Patents, China's latest patents, Technical Efficacy Thesaurus, Application Domain, Technology Topic, Popular Technical Reports.
© 2024 PatSnap. All rights reserved.Legal|Privacy policy|Modern Slavery Act Transparency Statement|Sitemap|About US| Contact US: help@patsnap.com