And searching reliable semi-supervised few-sample image classification method of abnormal data center
A technology of abnormal data and sample images, applied in instruments, biological neural network models, character and pattern recognition, etc., can solve problems such as unreasonableness, and achieve the effect of improving accuracy
- Summary
- Abstract
- Description
- Claims
- Application Information
AI Technical Summary
Problems solved by technology
Method used
Image
Examples
Embodiment Construction
[0022] The technical solutions of the present invention will be clearly and completely described below in conjunction with the accompanying drawings. Apparently, the described embodiments are some of the embodiments of the present invention, but not all of them. Based on the embodiments of the present invention, all other embodiments obtained by persons of ordinary skill in the art without making creative efforts belong to the protection scope of the present invention.
[0023] please see figure 1 , a semi-supervised few-sample image classification method for finding reliable abnormal data centers provided by the present invention, comprising the following steps:
[0024] Step 1: Divide the dataset;
[0025] Divide the dataset into training set D train , the test set D test , the training set and the test set contain different types of images, and the number of sample images of each type is not less than the preset value N;
[0026] In this embodiment, the data set is divi...
PUM
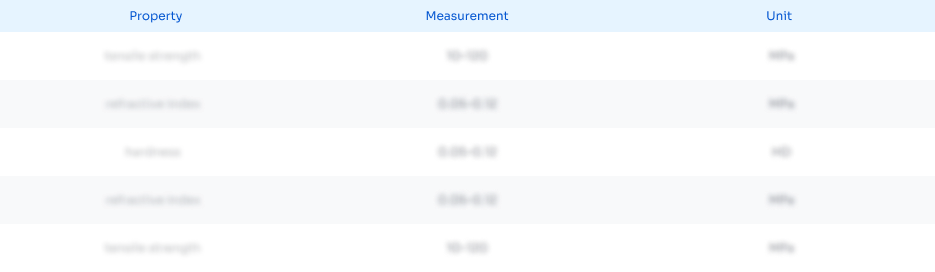
Abstract
Description
Claims
Application Information
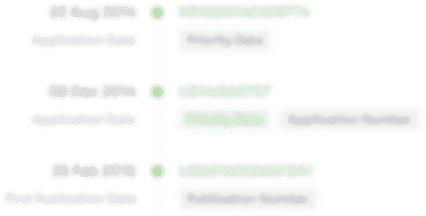
- R&D
- Intellectual Property
- Life Sciences
- Materials
- Tech Scout
- Unparalleled Data Quality
- Higher Quality Content
- 60% Fewer Hallucinations
Browse by: Latest US Patents, China's latest patents, Technical Efficacy Thesaurus, Application Domain, Technology Topic, Popular Technical Reports.
© 2025 PatSnap. All rights reserved.Legal|Privacy policy|Modern Slavery Act Transparency Statement|Sitemap|About US| Contact US: help@patsnap.com