Motor fault data enhancement method based on deep convolution generative adversarial network
A fault data, deep convolution technology, applied in the field of fault diagnosis and deep learning, can solve problems such as failure to detect and prevent motor faults in advance, inability to create conditions for conditional maintenance, and threats to personnel and equipment safety. Diversity, Prevention of Threats to Personnel and Equipment Safety, Effectiveness of Improved Accuracy
- Summary
- Abstract
- Description
- Claims
- Application Information
AI Technical Summary
Problems solved by technology
Method used
Image
Examples
Embodiment Construction
[0021] The present invention will be further invented in conjunction with the embodiments of the present invention below.
[0022] Please refer to Figure 1-2 , embodiment one:
[0023] The invention discloses a method for enhancing motor fault data based on a deep convolution generation confrontation network, the steps of which are as follows:
[0024] Step 1: First, classify and screen the real fault types. In the background, the fault types need to be classified into three fault types: damage fault, degeneration fault, and imbalance fault. The data of the fault types are placed in the fault Inside the type, in the process of data classification, the background can also delete missing and duplicate data to ensure the number of data is concise and the variety is rich, speed up the convergence of data, and reduce the data load of the background.
[0025] Step 2: Build a generative confrontation network, including two modules of generative model and discriminant model, which ...
PUM
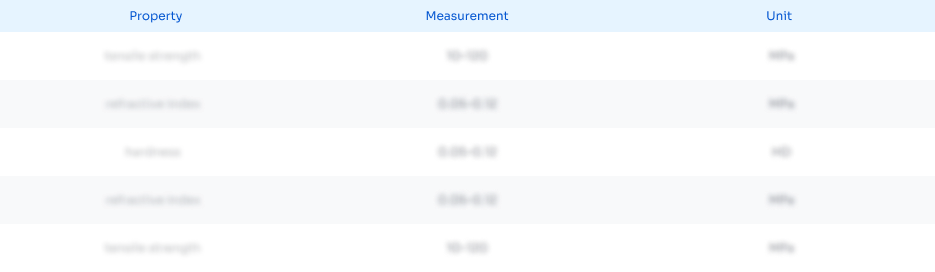
Abstract
Description
Claims
Application Information
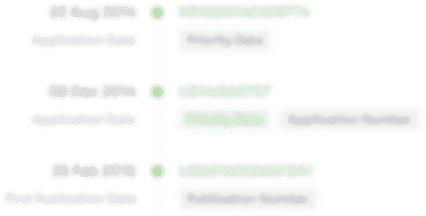
- R&D Engineer
- R&D Manager
- IP Professional
- Industry Leading Data Capabilities
- Powerful AI technology
- Patent DNA Extraction
Browse by: Latest US Patents, China's latest patents, Technical Efficacy Thesaurus, Application Domain, Technology Topic.
© 2024 PatSnap. All rights reserved.Legal|Privacy policy|Modern Slavery Act Transparency Statement|Sitemap