Data annotation, model training and image processing method and device and storage medium
An image and model technology, applied in the field of image processing, can solve the problems of missing labeling, difficulty in finding with naked eyes, and difficulty in labeling work.
- Summary
- Abstract
- Description
- Claims
- Application Information
AI Technical Summary
Problems solved by technology
Method used
Image
Examples
Embodiment 1
[0056] figure 2 A schematic block diagram of a data labeling method according to an embodiment of the present invention is shown.
[0057] Such as figure 2 As shown, according to the data labeling method of an embodiment of the present invention, this embodiment takes object detection in medical images as an example to illustrate the inventive solution, and the method of the present invention is not limited to the processing of medical images. In addition, in the embodiment of the present invention, the Faster R-CNN model is taken as an example to illustrate the neural network model used for target detection. The neural network model in the present invention is not limited to this, and various neural networks with different structures can be used in the present invention The model and the data labeled using the standard method of the present invention are trained to improve its detection effect on specific types of images and targets.
[0058] A sample data labeling method...
Embodiment 2
[0083] image 3 A schematic block diagram according to another embodiment of the present invention is shown.
[0084] Such as image 3 As shown, the second embodiment of the present invention provides a kind of training method for the neural network of object detection, and the neural network in the present embodiment generally comprises four parts: feature extraction network (convolutional layer), area generation network ( Region Proposal Network, RPN), target region pooling network (ROI pooling layer) and target classification network (Classification), typically, use the frame of Faster R-CNN model to construct the neural network of target detection in the present embodiment, Faster R -CNN model is an existing technology, a typical Faster R-CNN model is attached figure 1 As shown, the structure of an exemplary neural network in the present invention is briefly introduced below, and will not be elaborated again:
[0085] Among them, the feature extraction network or featur...
Embodiment 3
[0119] as attached Figure 5 As shown, Embodiment 3 of the present invention provides an image processing method using a neural network model to detect objects in an image. The method specifically includes the following steps:
[0120] S401: Acquire an image to be processed.
[0121] The image to be processed is an image of the same type as the sample image used for neural network training, and the target to be detected is the same as the target category marked in the sample image.
[0122] Same as the previous embodiment, this embodiment still takes the human lung image as an example, and the detection target is a lung nodule. The protection scope of the present invention is not limited thereto, and the data labeling method, neural network training method and image processing method of the present invention can be used to detect various types of images and targets according to different labeling data.
[0123] S402: Invoke a neural network model to perform target detection...
PUM
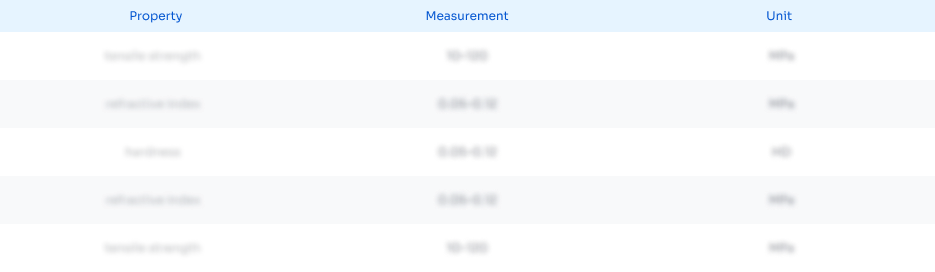
Abstract
Description
Claims
Application Information
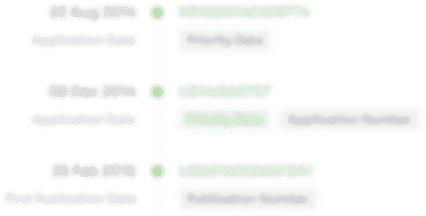
- R&D Engineer
- R&D Manager
- IP Professional
- Industry Leading Data Capabilities
- Powerful AI technology
- Patent DNA Extraction
Browse by: Latest US Patents, China's latest patents, Technical Efficacy Thesaurus, Application Domain, Technology Topic, Popular Technical Reports.
© 2024 PatSnap. All rights reserved.Legal|Privacy policy|Modern Slavery Act Transparency Statement|Sitemap|About US| Contact US: help@patsnap.com