Sample knowledge graph relationship learning method and system based on adversarial attention mechanism
A technology of knowledge graph and learning method, which is applied in the field of a sample knowledge graph relational learning method and system, which can solve the problems of few and difficult knowledge sharing and inductive transfer, so as to reduce noise, increase the difficulty of discrimination, and increase the relationship between classes The effect of discrimination
- Summary
- Abstract
- Description
- Claims
- Application Information
AI Technical Summary
Problems solved by technology
Method used
Image
Examples
Embodiment 1
[0028] In one or more embodiments, a method for learning a sample knowledge graph relationship based on an adversarial attention mechanism is disclosed, referring to figure 1 ,include:
[0029] Step 1: Obtain relational triples and their corresponding natural text descriptions in the target knowledge graph.
[0030] Step 2: Perform representation learning on the target knowledge graph to obtain the vector representation of triples.
[0031] The vector representation of each entity in the knowledge map is obtained by using the method based on representation learning. For the visible relationship r s a triplet of Get the vector representation x of the relation entity triplet i ,{i=1,2,3...T}.
[0032] Step 3: Perform representation learning on the corresponding text description of the triple to obtain the word vector representation in the text.
[0033] Use the word vector representation method to obtain the vector representation v of each word i ,{i=1,2,3...V}.
[0034]...
PUM
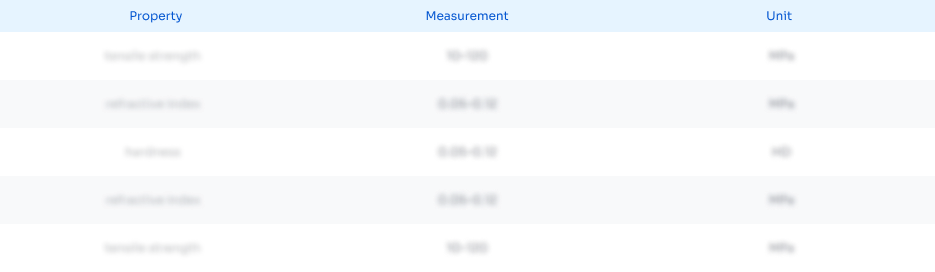
Abstract
Description
Claims
Application Information
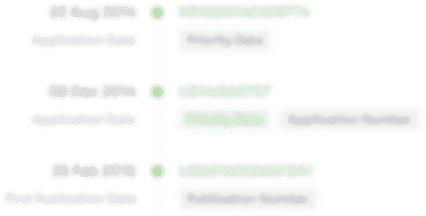
- R&D Engineer
- R&D Manager
- IP Professional
- Industry Leading Data Capabilities
- Powerful AI technology
- Patent DNA Extraction
Browse by: Latest US Patents, China's latest patents, Technical Efficacy Thesaurus, Application Domain, Technology Topic, Popular Technical Reports.
© 2024 PatSnap. All rights reserved.Legal|Privacy policy|Modern Slavery Act Transparency Statement|Sitemap|About US| Contact US: help@patsnap.com