Improved end-to-end speech recognition method
A speech recognition and speech technology, applied in speech recognition, speech analysis, instruments, etc., can solve the problems of complex construction process, time-consuming, inability to accurately represent speech signal distribution, etc., achieve excellent robustness, improve recognition rate and training efficiency effect
- Summary
- Abstract
- Description
- Claims
- Application Information
AI Technical Summary
Problems solved by technology
Method used
Image
Examples
Embodiment Construction
[0039] The present invention proposes an improved end-to-end speech recognition method, as attached figure 1 shown, including the following steps:
[0040] Step 1. Obtain the voice and its transcribed text data set, perform the feature extraction result of Mel Spectrum on the voice data as the input feature, and obtain the tag set and dictionary from the transcribed text.
[0041] Step 2. Build a model including a convolutional layer, a self-attention layer, and a fully connected layer. Use the CTC loss function as the loss function of the model, and use the backpropagation algorithm to update the model parameters.
[0042] Step 3. Using the trained model, the speech feature sequence is used as an input to obtain an output, and the output result is decoded to obtain a final result.
[0043] The following is a detailed description with reference to the illustrations.
[0044] Firstly, the speech feature data and its transcription text labels are obtained by using the speech a...
PUM
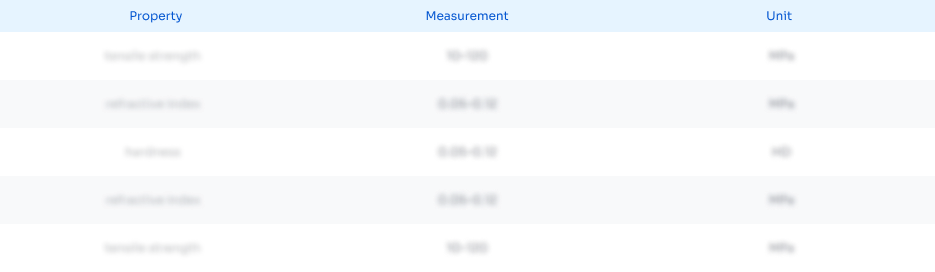
Abstract
Description
Claims
Application Information
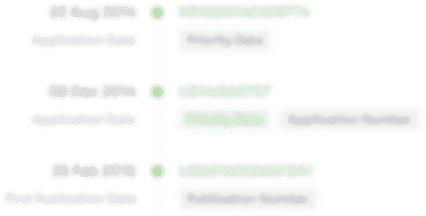
- R&D Engineer
- R&D Manager
- IP Professional
- Industry Leading Data Capabilities
- Powerful AI technology
- Patent DNA Extraction
Browse by: Latest US Patents, China's latest patents, Technical Efficacy Thesaurus, Application Domain, Technology Topic, Popular Technical Reports.
© 2024 PatSnap. All rights reserved.Legal|Privacy policy|Modern Slavery Act Transparency Statement|Sitemap|About US| Contact US: help@patsnap.com