Weak supervision video sequential action positioning method and system based on deep learning
A technology of deep learning and positioning method, applied in the field of computer vision based on deep learning, can solve the problems of ignoring action semantic consistency, not explicitly modeling action semantic consistency, and uncontrollable training process.
- Summary
- Abstract
- Description
- Claims
- Application Information
AI Technical Summary
Problems solved by technology
Method used
Image
Examples
Embodiment Construction
[0037] The implementation of the present invention is described below through specific examples and in conjunction with the accompanying drawings, and those skilled in the art can easily understand other advantages and effects of the present invention from the content disclosed in this specification. The present invention can also be implemented or applied through other different specific examples, and various modifications and changes can be made to the details in this specification based on different viewpoints and applications without departing from the spirit of the present invention.
[0038] figure 1It is a flow chart of the steps of a weakly supervised video sequence action location method based on deep learning in the present invention, figure 2 It is a schematic diagram of the deep learning-based weakly supervised video time-series action location process according to a specific embodiment of the present invention. Such as figure 1 and figure 2 As shown, the pres...
PUM
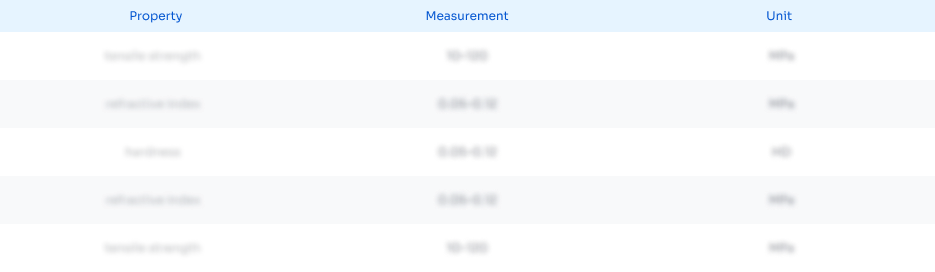
Abstract
Description
Claims
Application Information
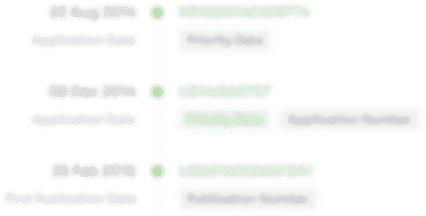
- R&D
- Intellectual Property
- Life Sciences
- Materials
- Tech Scout
- Unparalleled Data Quality
- Higher Quality Content
- 60% Fewer Hallucinations
Browse by: Latest US Patents, China's latest patents, Technical Efficacy Thesaurus, Application Domain, Technology Topic, Popular Technical Reports.
© 2025 PatSnap. All rights reserved.Legal|Privacy policy|Modern Slavery Act Transparency Statement|Sitemap|About US| Contact US: help@patsnap.com