Sequence recommendation method based on self-attention auto-encoder
A technology of autoencoder and recommendation method, applied in the direction of neural learning methods, instruments, complex mathematical operations, etc., can solve the problems of low accuracy and inability to consider users' long-term and short-term preferences at the same time
- Summary
- Abstract
- Description
- Claims
- Application Information
AI Technical Summary
Problems solved by technology
Method used
Image
Examples
Embodiment 1
[0089] Such as figure 1 As shown, the sequence recommendation method based on self-attention autoencoder includes the following steps:
[0090] S1: Obtain the user product sequence and scoring matrix, and preprocess the product sequence;
[0091] S2: Use the self-attention model to train the product sequence and predict the correlation score between the user and the product;
[0092] S3: Use the autoencoder to reconstruct the scoring matrix and calculate the user preference index;
[0093] S4: Combining the correlation score between the user and the product and the user preference index, the product with a high score is recommended for the user first.
[0094] More specifically, in the step S1, the process of preprocessing the commodity sequence is as follows:
[0095] The product sequence S browsed by user u u split into input sequences (S 1 ,S 2 ,...,S |su-1| ) and the output sequence (S 2 ,S 3 ,...,S |su| ), where the input sequence is used a...
Embodiment 2
[0161] More specifically, the present invention proposes a sequence recommendation method based on a self-attention autoencoder, which uses a self-attention mechanism to model the interaction between the user's long and short preferences, and adds an autoencoder to The user's rating matrix is reconstructed, and then the information of these two modules is fused, so as to realize the personalized intelligent recommendation for the user. Such as figure 2 As shown, the overall frame diagram of the algorithm of the present invention mainly includes two parts: the self-attention model and the autoencoder model.
[0162] In the specific implementation process, such as image 3 The word vector learning neural network structure of the embedding layer of the self-attention model shown above, the idea of the neural network work is based on the distributed assumption, that is, two identical commodities, if their contexts are similar, then the two commodity It may also be a h...
Embodiment 3
[0211] More specifically, the three data sets of the beauty makeup (Beauty) data set and the video game (Videogame) data set in the Amazon data source, and the MovieLens1M data set in the MovieLens data source are selected to evaluate the method of the present invention. The specific information of the set is shown in Table 1:
[0212] Table 1
[0213]
[0214] Two sets of evaluation metrics were used in this experiment. For the self-encoder, the mean square error is used as the evaluation index; for the self-attention model and the entire model of the present invention, the two most common Top k evaluation indexes are selected, which are respectively hit rate (Hitrate@k) and normalized discounted cumulative gain (NDCG@k).
[0215] The parameter settings of this experiment are slightly different on the three datasets. In the selection of several important parameters, the final model parameter values are selected by comparing the performance of the model on d...
PUM
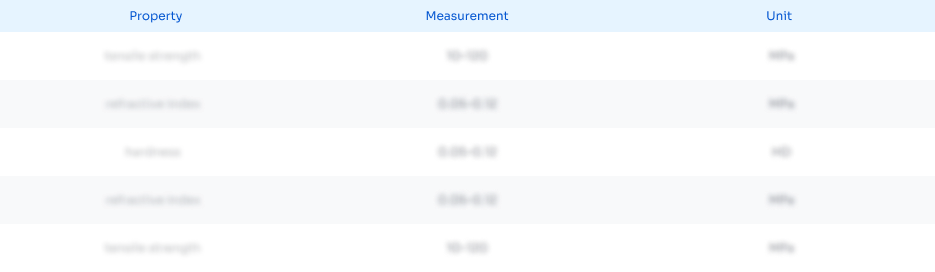
Abstract
Description
Claims
Application Information
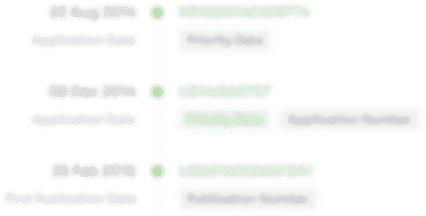
- Generate Ideas
- Intellectual Property
- Life Sciences
- Materials
- Tech Scout
- Unparalleled Data Quality
- Higher Quality Content
- 60% Fewer Hallucinations
Browse by: Latest US Patents, China's latest patents, Technical Efficacy Thesaurus, Application Domain, Technology Topic, Popular Technical Reports.
© 2025 PatSnap. All rights reserved.Legal|Privacy policy|Modern Slavery Act Transparency Statement|Sitemap|About US| Contact US: help@patsnap.com