Image semantic segmentation method based on context and shallow space coding and decoding network
A semantic segmentation and context technology, applied in the field of computer vision and deep learning, can solve the problems of not obtaining high-quality semantic context features, ignoring the shallow spatial details of the network, etc.
- Summary
- Abstract
- Description
- Claims
- Application Information
AI Technical Summary
Problems solved by technology
Method used
Image
Examples
Embodiment
[0053] In order to verify the effectiveness of the proposed module, the following ablation experiments were performed on the CamVid dataset. The present invention uses four schemes to evaluate the performance of the hybrid expansion module and the residual pyramid feature extraction module: (1) The context path at the encoding end uses only the hybrid expansion convolution module; (2) the context path at the encoding end uses only the residual pyramid feature Extraction module; (3) There is no hybrid expansion convolution and residual pyramid feature extraction module on the encoding side context path; (4) Hybrid expansion convolution module and residual pyramid feature extraction module are used on the encoding side context path. The experimental results are shown in Table 1. It can be seen from the table that the best segmentation performance is obtained when the hybrid expansion convolution module and the residual pyramid feature extraction module are used at the same time, i...
PUM
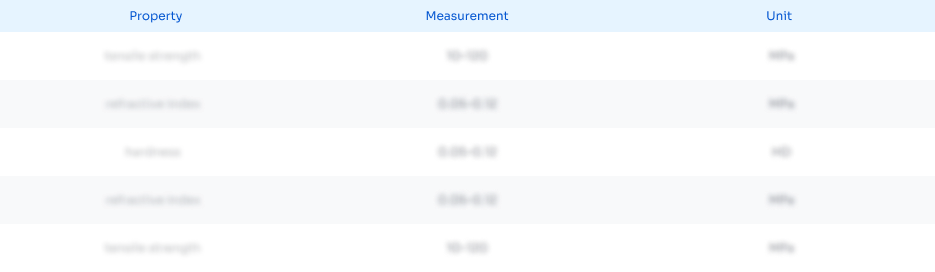
Abstract
Description
Claims
Application Information
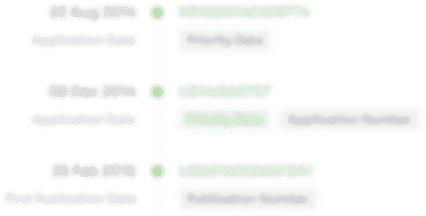
- R&D
- Intellectual Property
- Life Sciences
- Materials
- Tech Scout
- Unparalleled Data Quality
- Higher Quality Content
- 60% Fewer Hallucinations
Browse by: Latest US Patents, China's latest patents, Technical Efficacy Thesaurus, Application Domain, Technology Topic, Popular Technical Reports.
© 2025 PatSnap. All rights reserved.Legal|Privacy policy|Modern Slavery Act Transparency Statement|Sitemap|About US| Contact US: help@patsnap.com