Cross-modal medical image synthesis method based on parallel generative network
A synthesis method and technology of medical images, applied in image enhancement, image analysis, image data processing and other directions, can solve problems such as difficult to achieve, lack of training data and accurate understanding of related experimental phenomena.
- Summary
- Abstract
- Description
- Claims
- Application Information
AI Technical Summary
Problems solved by technology
Method used
Image
Examples
Embodiment Construction
[0044] The present invention will be further described below in conjunction with the accompanying drawings.
[0045] refer to figure 1 and figure 2 , a method for cross-modal medical image synthesis based on parallel generative networks, comprising the following steps:
[0046] 1) Establish the basis for grouping based on expert experience, and use the small sample training set of the corresponding modality to classify the CNN-based grouper C A 、C B For training, the process is as follows:
[0047] 1.1) According to expert experience, specify groups 1, 2, 3...n of the MR data set, a total of n groups of representative images (several pictures in each group), where the feature of each group is the space represented by the image Anatomical structure, CT reference map obtained in the same way as above;
[0048] 1.2) Use the training set of MR to group the C A For training, use the training set of CT to group the C B Carrying out training, the grouper performs group proba...
PUM
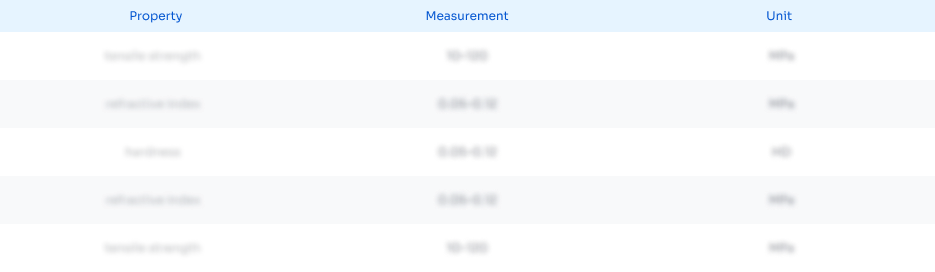
Abstract
Description
Claims
Application Information
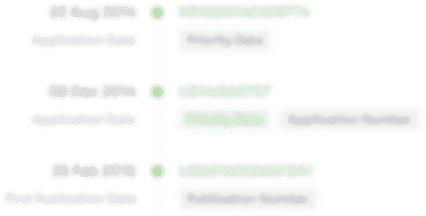
- R&D Engineer
- R&D Manager
- IP Professional
- Industry Leading Data Capabilities
- Powerful AI technology
- Patent DNA Extraction
Browse by: Latest US Patents, China's latest patents, Technical Efficacy Thesaurus, Application Domain, Technology Topic, Popular Technical Reports.
© 2024 PatSnap. All rights reserved.Legal|Privacy policy|Modern Slavery Act Transparency Statement|Sitemap|About US| Contact US: help@patsnap.com