Target tracking method based on residual dense twin network
A twin network and target tracking technology, which is applied in the fields of image processing and computer vision, can solve problems such as inability to accurately locate targets, similar semantic interference, and inability to deal with target tracking background clutter.
- Summary
- Abstract
- Description
- Claims
- Application Information
AI Technical Summary
Problems solved by technology
Method used
Image
Examples
Embodiment Construction
[0059] The present invention provides a target tracking method based on the residual dense Siamese network. The method first extracts the template image of the target to be tracked in the first frame image of the video, and inputs it into the residual dense network to obtain the initial template features. The extracted features are further input into the global attention module to obtain the template features, and the tracker initialization is completed; then the t-th frame image is cropped to extract the search area image, and input to the residual dense network to obtain the search area features; finally, the template The features and search area features are input into the candidate area generation network to obtain the foreground and background classification confidence and bounding box regression estimates, and further obtain the tracking result of the tth frame. Application of the present invention solves the problem that the existing twin network-based target tracking me...
PUM
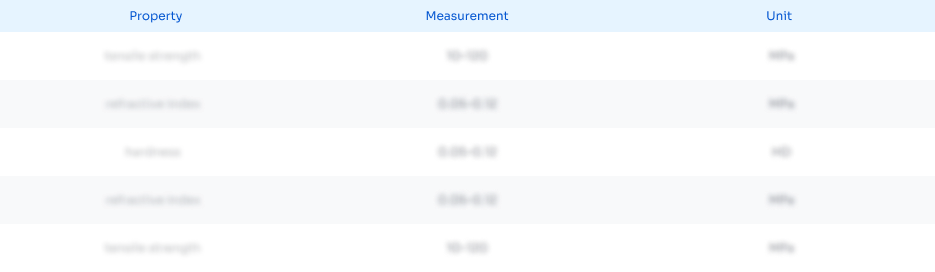
Abstract
Description
Claims
Application Information
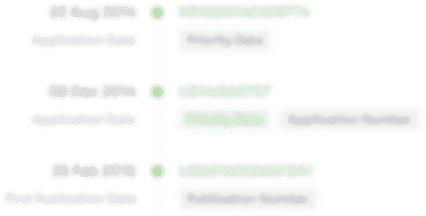
- R&D Engineer
- R&D Manager
- IP Professional
- Industry Leading Data Capabilities
- Powerful AI technology
- Patent DNA Extraction
Browse by: Latest US Patents, China's latest patents, Technical Efficacy Thesaurus, Application Domain, Technology Topic.
© 2024 PatSnap. All rights reserved.Legal|Privacy policy|Modern Slavery Act Transparency Statement|Sitemap