Method for constructing missing sample probability density function estimator
A technique of probability density function and estimator, applied in nonlinear wavelet estimation, constructing the field of nonlinear wavelet estimator of probability density function of missing samples, which can solve the problem of inability to estimate non-smooth probability density function, so as to reduce deviation and improve effectiveness. Effect
- Summary
- Abstract
- Description
- Claims
- Application Information
AI Technical Summary
Problems solved by technology
Method used
Image
Examples
Embodiment Construction
[0053] With reference to accompanying drawing, further illustrate the present invention:
[0054] A method for constructing an estimator of a missing-sample probability density function comprising the steps of:
[0055] (1), using wavelet basis functions and wavelet coefficients to expand the real probability density function into wavelet form;
[0056] Suppose {X i ,1≤i≤n} is a d-dimensional covariate, Y i is affected by the covariate X i Affects the response variable, and has a probability density function f(y), for any f(y)∈L 2 (R) space can be expanded into the following wavelet form
[0057]
[0058] in, and beta kl =kl , f> is the wavelet coefficient, Form L 2 A set of orthonormal basis for (R) space. In order to study the convergence of the probability density function estimator, it is necessary to give k a truncation k 1 , so the probability density function can be expanded into the following form
[0059]
[0060] The probability density function f(...
PUM
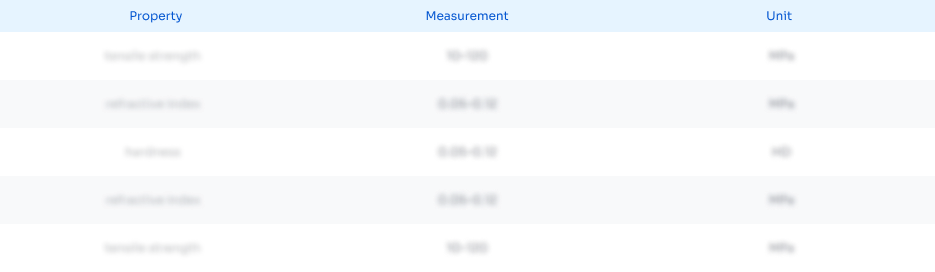
Abstract
Description
Claims
Application Information
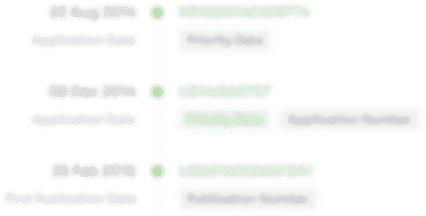
- R&D Engineer
- R&D Manager
- IP Professional
- Industry Leading Data Capabilities
- Powerful AI technology
- Patent DNA Extraction
Browse by: Latest US Patents, China's latest patents, Technical Efficacy Thesaurus, Application Domain, Technology Topic, Popular Technical Reports.
© 2024 PatSnap. All rights reserved.Legal|Privacy policy|Modern Slavery Act Transparency Statement|Sitemap|About US| Contact US: help@patsnap.com