A method of object detection based on fully automatic learning
A target detection, fully automatic technology, applied in the field of target detection based on automatic learning, can solve the problems of high labor cost, poor transferability and adaptability of training models, etc., to ensure effectiveness and efficiency, and improve rapid adaptability , the effect of reducing the likelihood
- Summary
- Abstract
- Description
- Claims
- Application Information
AI Technical Summary
Problems solved by technology
Method used
Image
Examples
Embodiment Construction
[0029] In order to make the purpose, technical solution and advantages of the present invention clearer, the implementation manners of the present invention will be further described in detail below.
[0030] In the image recognition scenario, based on active learning and self-supervision, this method proposes a fully automatic learning method for target detection, which solves the cost problem of data labeling and model category migration, and ensures that the model can well adapt to the detection task in practical applications.
[0031] Such as Figure 1-3 Shown, concrete steps of the present invention are:
[0032] 1. Data preparation stage
[0033] Firstly, construct a large-scale original image data set, screen out meaningless pictures through preprocessing, obtain the overall information of the data set, obtain the training set, verification set and test set, divide it into k sets of data, and manually perform a set of data analysis For initial annotation, normalize th...
PUM
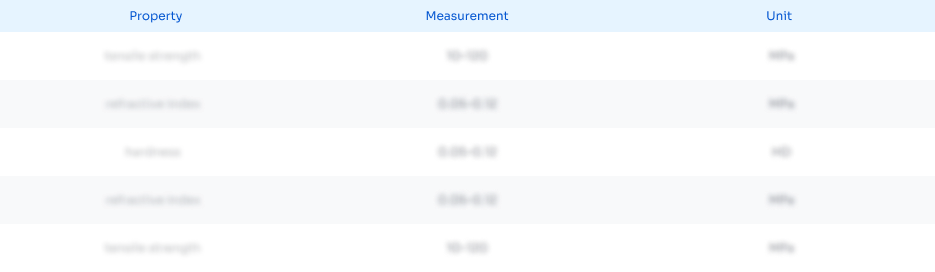
Abstract
Description
Claims
Application Information
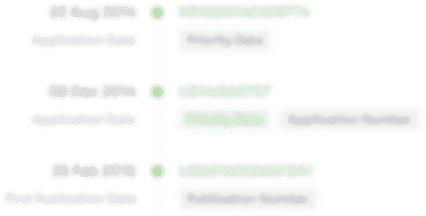
- R&D
- Intellectual Property
- Life Sciences
- Materials
- Tech Scout
- Unparalleled Data Quality
- Higher Quality Content
- 60% Fewer Hallucinations
Browse by: Latest US Patents, China's latest patents, Technical Efficacy Thesaurus, Application Domain, Technology Topic, Popular Technical Reports.
© 2025 PatSnap. All rights reserved.Legal|Privacy policy|Modern Slavery Act Transparency Statement|Sitemap|About US| Contact US: help@patsnap.com