Image incremental learning method based on dynamic correction vector
A technique for dynamic correction, learning methods, applied in the fields of knowledge distillation techniques and representational memory
- Summary
- Abstract
- Description
- Claims
- Application Information
AI Technical Summary
Problems solved by technology
Method used
Image
Examples
Embodiment Construction
[0044] The present invention will be further described below in conjunction with the accompanying drawings of the description.
[0045] refer to Figure 1 ~ Figure 3 , an image incremental learning method based on dynamic correction vectors, which solves the problem of deep model training on dynamically changing data sets, reduces the dependence on distributed computing systems, and saves a lot of computing overhead and system memory. The invention proposes to use the 32-layer residual network ResNet-32 as the basis, introduce knowledge distillation technology and representative memory method, and use the technique of dynamic correction vector to alleviate the problem of catastrophic forgetting and improve the performance of incremental learning.
[0046] The present invention comprises the following steps:
[0047] S1: Construct a backbone network modeled on the ResNet-32 network layer structure to identify new and old categories that appear in the incremental phase tasks. T...
PUM
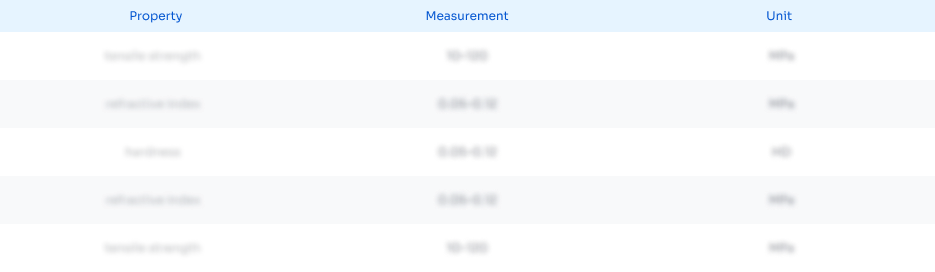
Abstract
Description
Claims
Application Information
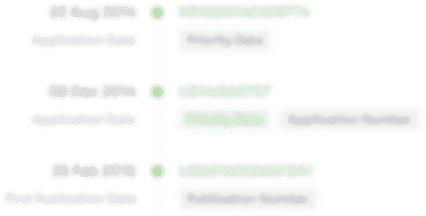
- R&D Engineer
- R&D Manager
- IP Professional
- Industry Leading Data Capabilities
- Powerful AI technology
- Patent DNA Extraction
Browse by: Latest US Patents, China's latest patents, Technical Efficacy Thesaurus, Application Domain, Technology Topic.
© 2024 PatSnap. All rights reserved.Legal|Privacy policy|Modern Slavery Act Transparency Statement|Sitemap