Action real-time monitoring method based on YOLO
A real-time monitoring and action technology, applied in the direction of instruments, character and pattern recognition, computer components, etc., can solve the problems of inaccurate detection, single detection method, poor robustness of segmentation effect, etc., and achieve the effect of ensuring efficiency
- Summary
- Abstract
- Description
- Claims
- Application Information
AI Technical Summary
Problems solved by technology
Method used
Image
Examples
Embodiment 1
[0044]Embodiment 1: A method for real-time monitoring of actions based on YOLO. The monitoring method includes the following steps: S1: Establish a scene model, and the scene model is configured to collect video at the position of the camera; S2: Perform a video capture on the scene Object detection in the model to obtain the detection frame; S3: Construct and train the deep learning extraction network, input the detection frames of all people into the network, and obtain the feature vectors of all detection frames; S4: Predict the action vector of the video tracking target, Use the feature vector of the detection frame and the predicted action vector to match or predict the dangerous action of the tracking target to obtain the best matching and predicted detection frame; S5: Red mark the detection frame of the dangerous action; S6: Alarm prompt. In this embodiment, the camera adopts a 〖YOLOv〗_3 camera to ensure real-time monitoring of the scene and accurate identification of d...
Embodiment 2
[0045] Embodiment 2: A method for real-time monitoring of actions based on YOLO, the monitoring method includes the following steps: S1: establish a scene model, and the scene model is configured to collect video at the position of the camera; S2: perform a video capture on the scene Object detection in the model to obtain the detection frame; S3: Construct and train the deep learning extraction network, input the detection frames of all people into the network, and obtain the feature vectors of all detection frames; S4: Predict the action vector of the video tracking target, Use the feature vector of the detection frame and the predicted action vector to match or predict the dangerous action of the tracking target to obtain the best matching and predicted detection frame; S5: Red mark the detection frame of the dangerous action; S6: Alarm prompt. Specifically, the present invention discloses a method for real-time monitoring of actions based on YOLO, mainly for the purpose of ...
PUM
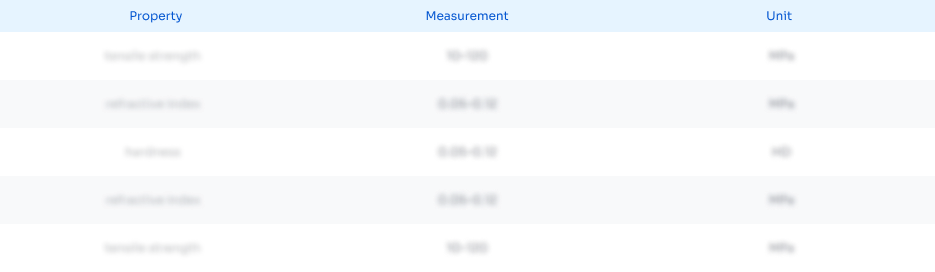
Abstract
Description
Claims
Application Information
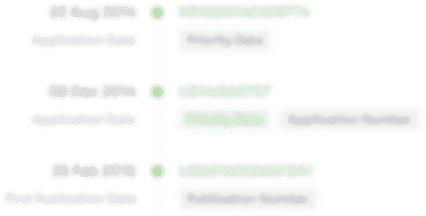
- R&D Engineer
- R&D Manager
- IP Professional
- Industry Leading Data Capabilities
- Powerful AI technology
- Patent DNA Extraction
Browse by: Latest US Patents, China's latest patents, Technical Efficacy Thesaurus, Application Domain, Technology Topic.
© 2024 PatSnap. All rights reserved.Legal|Privacy policy|Modern Slavery Act Transparency Statement|Sitemap