Single-point intersection signal control method based on deep cyclic Q learning
A signal control, single-point intersection technology, applied in the field of single-point intersection signal control based on deep cyclic Q-learning, can solve problems such as affecting algorithm performance, different real-time state values and real values, and inaccurate sensors, achieving improved performance. The effect of traffic efficiency
- Summary
- Abstract
- Description
- Claims
- Application Information
AI Technical Summary
Problems solved by technology
Method used
Image
Examples
Embodiment Construction
[0037] The application will be further described in detail below in conjunction with the accompanying drawings and embodiments. It should be understood that the specific embodiments described here are only used to explain related inventions, not to limit the invention. It should also be noted that, for the convenience of description, only the parts related to the related invention are shown in the drawings.
[0038] A single-point intersection signal control method based on deep cycle Q learning, the main flow chart is as follows figure 1 , including the following steps:
[0039] Step 1: Determine the intersection that needs to be optimally controlled, and obtain the traffic video collection data of the intersection for a period of time. The data source used in this embodiment is the video data at the intersection of Liyuan South Road and Gaohu Road, and the position of the video coil is as follows figure 2 As shown, the format of the video data is parsed as follows:
[0...
PUM
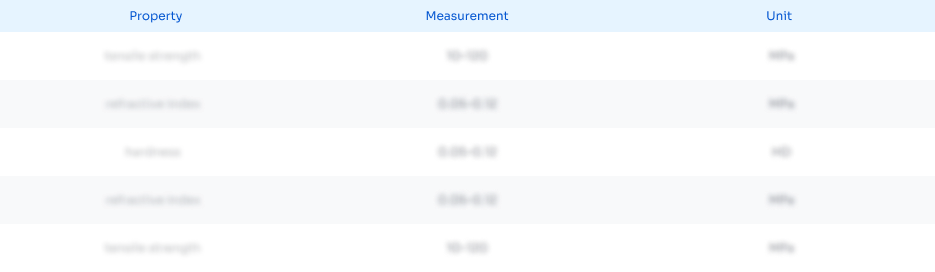
Abstract
Description
Claims
Application Information
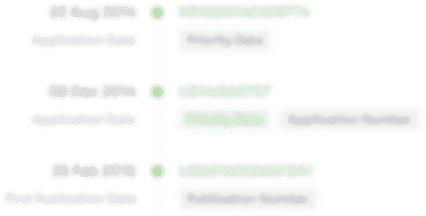
- R&D
- Intellectual Property
- Life Sciences
- Materials
- Tech Scout
- Unparalleled Data Quality
- Higher Quality Content
- 60% Fewer Hallucinations
Browse by: Latest US Patents, China's latest patents, Technical Efficacy Thesaurus, Application Domain, Technology Topic, Popular Technical Reports.
© 2025 PatSnap. All rights reserved.Legal|Privacy policy|Modern Slavery Act Transparency Statement|Sitemap|About US| Contact US: help@patsnap.com