Category label recovery method for low-rank image feature analysis
A technology of category labels and image features, applied in character and pattern recognition, instruments, computer parts, etc., can solve the problems of model underfitting, missing labels, and low recognition rate.
- Summary
- Abstract
- Description
- Claims
- Application Information
AI Technical Summary
Problems solved by technology
Method used
Image
Examples
Embodiment Construction
[0017] In order to make the present invention more obvious and understandable, the preferred embodiments are described in detail as follows in conjunction with the accompanying drawings:
[0018] Such as figure 1 As shown, first obtain all samples of the original sample set A, a total of n sample images, a total of C categories, in the c category there are N e samples, c ∈ {1, 2, ..., C}. The size of each image is S×S pixels, and the S×S-dimensional image matrix is pulled into an S 2 dimensional column vector, n images form a S 2 ×n-dimensional sample matrix x=[x 1 , x 2 ,...,x n ], the label y corresponding to each image 1 ,...y n ∈ {1, 2, ..., C}, corresponding to the original label matrix Y = [y 1 ,y 2 ,...,y n ]. For the above-mentioned original sample set A, the present invention provides a method for recovering class labels for low-rank image feature analysis, comprising the following steps:
[0019] Step 1: Generate u non-repetitive random numbers, and in ...
PUM
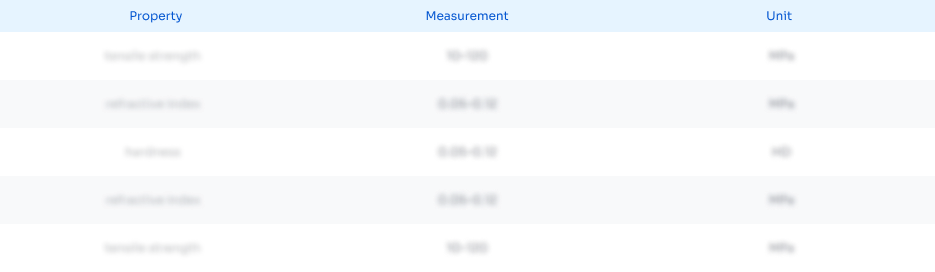
Abstract
Description
Claims
Application Information
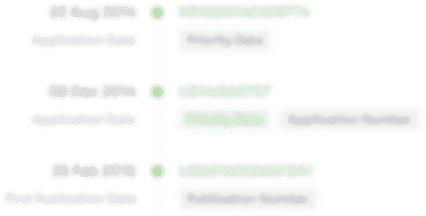
- R&D
- Intellectual Property
- Life Sciences
- Materials
- Tech Scout
- Unparalleled Data Quality
- Higher Quality Content
- 60% Fewer Hallucinations
Browse by: Latest US Patents, China's latest patents, Technical Efficacy Thesaurus, Application Domain, Technology Topic, Popular Technical Reports.
© 2025 PatSnap. All rights reserved.Legal|Privacy policy|Modern Slavery Act Transparency Statement|Sitemap|About US| Contact US: help@patsnap.com