Unsupervised absolute scale calculation method and system
A calculation method and unsupervised technology, applied in the field of computer vision, can solve the problems of scale uncertainty, the inability to obtain the true value of each frame image, and the difficulty of applying scale restoration methods to achieve the effect of ensuring structural consistency
- Summary
- Abstract
- Description
- Claims
- Application Information
AI Technical Summary
Problems solved by technology
Method used
Image
Examples
Embodiment 1
[0053] Such as Figure 1-9 As shown, a method and system for unsupervised absolute scale calculation, in which the following modules are mainly used: pose estimation depth network module T, depth estimation depth network module G1, depth network module G2 for restoring a reference RGB image according to a reference depth map, Discrimination module D1, discrimination module D2 and error loss function module. Module T includes encoder and predictor, module G1, module G2, module D1 and module D2 all include encoder and decoder, the encoder of module T adopts ResNet18 network structure, and the predictor of module T adopts Figure 8 As shown in the structure, the predictor is a network structure composed of 4 convolutional layers; the encoder of module G1 adopts ResNet18 network structure, and the decoder of module G1 adopts Figure 7 As shown in the structure, the decoder is a network structure composed of 5 layers of deconvolution layers; the encoder of module G2 adopts the Res...
PUM
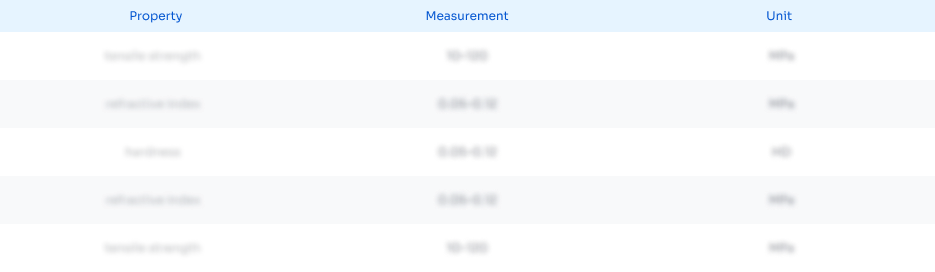
Abstract
Description
Claims
Application Information
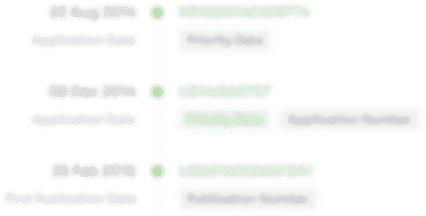
- R&D Engineer
- R&D Manager
- IP Professional
- Industry Leading Data Capabilities
- Powerful AI technology
- Patent DNA Extraction
Browse by: Latest US Patents, China's latest patents, Technical Efficacy Thesaurus, Application Domain, Technology Topic, Popular Technical Reports.
© 2024 PatSnap. All rights reserved.Legal|Privacy policy|Modern Slavery Act Transparency Statement|Sitemap|About US| Contact US: help@patsnap.com