Hybrid recommendation method based on user commodity portrait and potential factor feature extraction
A feature extraction and mixed recommendation technology, applied in the field of artificial intelligence, can solve the problems of sparse features, reduced recommendation efficiency, and inability to make recommendations, so as to improve robustness and avoid the effect of dimensionality disaster
- Summary
- Abstract
- Description
- Claims
- Application Information
AI Technical Summary
Problems solved by technology
Method used
Image
Examples
Embodiment 1
[0093] Embodiment one: adopt the data set of Movielens1M size to verify the method that the present invention proposes, and experimental process is as follows:
[0094] (1) Data preprocessing: preprocess the three files in the data set, the data includes user data set, movie data set and rating data set. The user data set contains user ID, gender, age group, occupation and other information. The movie dataset contains movie ID, movie showtime, movie title, and movie genre. The Ratings dataset contains user rating data for movies. The data processing method is as follows: For the discrete variables of gender, age group, and occupation in the user data, encode them according to Step1.2; for the movie title in the movie data, perform feature extraction according to the steps in Step1.3, and summarize the user and Feature representation after movie processing. Normalize the overall features.
[0095] (2) Construct the user's rating matrix for movies based on the rating data se...
Embodiment 2
[0103] Embodiment 2: Use the completion status of developers on a certain bidding website crawled by web crawlers to recommend reasonable developers for each task according to the method of the present invention. The experimental process is as follows:
[0104] (1) Data preprocessing: the data set includes developer information data, task data, and developer score data on task completion. The developer data set includes developer ID, developer skill label, developer task completion status, etc. , the task dataset contains information such as task ID, skill tags involved in the task, and submission time. The score dataset contains the scores of different developers for each task. Clean the data set and filter useful information. The features of different fields are encoded using the Step1 processing method, and the feature representations processed by the task and the developer are respectively summarized. Normalize the overall features.
[0105] (2) Construct the task and ...
PUM
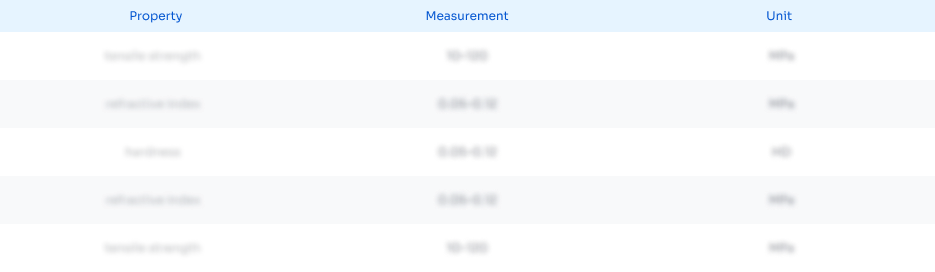
Abstract
Description
Claims
Application Information
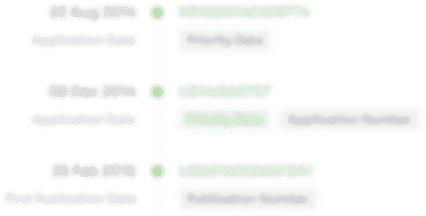
- R&D
- Intellectual Property
- Life Sciences
- Materials
- Tech Scout
- Unparalleled Data Quality
- Higher Quality Content
- 60% Fewer Hallucinations
Browse by: Latest US Patents, China's latest patents, Technical Efficacy Thesaurus, Application Domain, Technology Topic, Popular Technical Reports.
© 2025 PatSnap. All rights reserved.Legal|Privacy policy|Modern Slavery Act Transparency Statement|Sitemap|About US| Contact US: help@patsnap.com