Compressed representation learning method based on tensor decomposition
A learning method and tensor decomposition technology, applied in the direction of neural learning methods, instruments, biological neural network models, etc., can solve the redundancy or deficiency of downstream tasks, it is difficult to migrate to specific supervision tasks, and stay in small data set reasoning tasks And other issues
- Summary
- Abstract
- Description
- Claims
- Application Information
AI Technical Summary
Problems solved by technology
Method used
Image
Examples
Embodiment Construction
[0057] Below in conjunction with accompanying drawing, further describe the present invention through embodiment, but do not limit the scope of the present invention in any way.
[0058] This invention shows how representation learning can be enhanced with differentiable programmable tools. To encode a prior about the representation itself, that good representations should be compact and cohesive, we model the representation directly. Through visualization, we found that the first idea in the background technology is based on the insufficiency of the representation learned through pre-training, which often manifests in two types: missing and redundant. Considering that the classic tensor decomposition model is often used for image completion and denoising, we hope that this type of model can solve the lack and redundancy of pre-trained representations, and then establish differentiable tensors on the pre-trained representations. Quantitative decomposition model. The inventio...
PUM
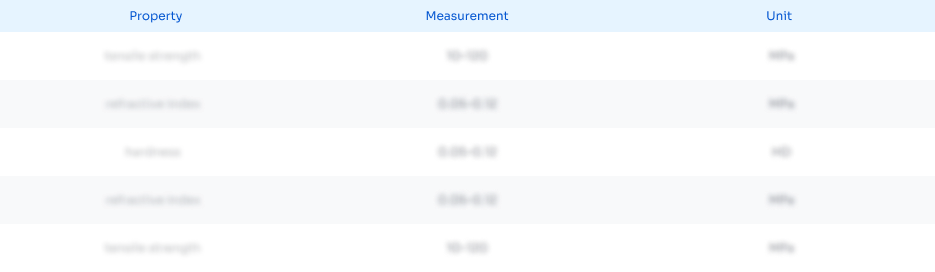
Abstract
Description
Claims
Application Information
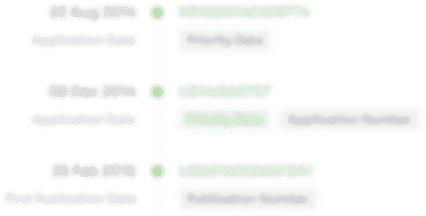
- R&D
- Intellectual Property
- Life Sciences
- Materials
- Tech Scout
- Unparalleled Data Quality
- Higher Quality Content
- 60% Fewer Hallucinations
Browse by: Latest US Patents, China's latest patents, Technical Efficacy Thesaurus, Application Domain, Technology Topic, Popular Technical Reports.
© 2025 PatSnap. All rights reserved.Legal|Privacy policy|Modern Slavery Act Transparency Statement|Sitemap|About US| Contact US: help@patsnap.com