RGB-D image semantic segmentation method based on multi-modal adaptive convolution
A RGB-D, semantic segmentation technology, applied in the field of image semantic segmentation and deep learning, can solve the problem of low accuracy and achieve the effect of improving the accuracy of semantic segmentation
- Summary
- Abstract
- Description
- Claims
- Application Information
AI Technical Summary
Problems solved by technology
Method used
Image
Examples
Embodiment Construction
[0029] In order to make the object, technical solution and advantages of the present invention clearer, the present invention will be described in further detail below in combination with specific implementation and accompanying drawings.
[0030] Such as figure 1 As shown, a RGB-D image semantic segmentation method based on multimodal adaptive convolution provided by the embodiment of the present invention, figure 2 For the specific structure of the RGB-D image semantic segmentation model based on multimodal adaptive convolution proposed by the present invention, it mainly includes the following steps:
[0031] 1) Send the paired RGB image and depth image into the encoding module, and use two identical encoding branches to extract the RGB features and depth features of the image, as follows:
[0032] Use the encoding module to extract the RGB features of the RGB image and the depth features of the depth image. The encoding module is a double-branch network, and each branch ...
PUM
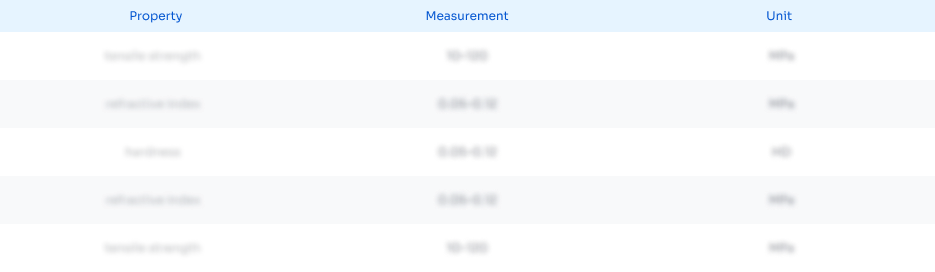
Abstract
Description
Claims
Application Information
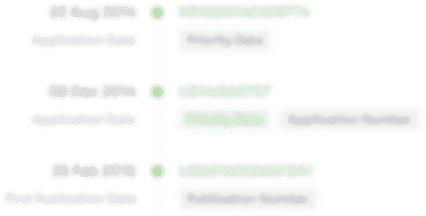
- R&D Engineer
- R&D Manager
- IP Professional
- Industry Leading Data Capabilities
- Powerful AI technology
- Patent DNA Extraction
Browse by: Latest US Patents, China's latest patents, Technical Efficacy Thesaurus, Application Domain, Technology Topic, Popular Technical Reports.
© 2024 PatSnap. All rights reserved.Legal|Privacy policy|Modern Slavery Act Transparency Statement|Sitemap|About US| Contact US: help@patsnap.com