Multi-scale feature optical flow learning calculation method based on self-attention mechanism
A technology of multi-scale features and calculation methods, which is applied in computing, image data processing, instruments, etc., can solve problems such as motion boundary blur, and achieve the effect of improving the boundary blur phenomenon
- Summary
- Abstract
- Description
- Claims
- Application Information
AI Technical Summary
Problems solved by technology
Method used
Image
Examples
Embodiment Construction
[0029] The present invention will be further described below in conjunction with drawings and embodiments. see Figure 1a to Figure 5 , a multi-scale feature optical flow learning calculation method based on the self-attention mechanism, using the Temple3 image sequence optical flow calculation experiment to illustrate:
[0030] 1) input Figure 1a and Figure 1b It is two consecutive frames of images in the Temple3 image sequence; where: Figure 1a is the first frame image, Figure 1b is the second frame image, corresponding to a resolution of 448×512;
[0031] 2) Carry out K=6 layer pyramid feature extraction to the selected two frames of images, and solve the initial optical flow field of the sequence, the initial optical flow field is as follows figure 2 shown;
[0032] 3) Use 3×3 convolution operation to perform feature fusion on the initial optical flow field and its corresponding features, and down-sample the fused features to match the size of the optical flow fea...
PUM
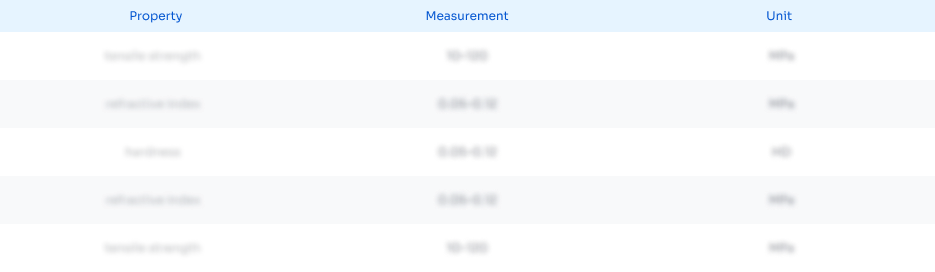
Abstract
Description
Claims
Application Information
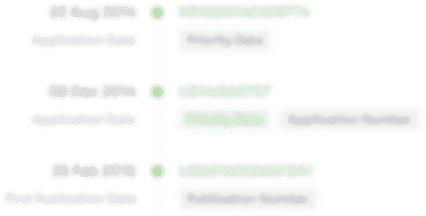
- Generate Ideas
- Intellectual Property
- Life Sciences
- Materials
- Tech Scout
- Unparalleled Data Quality
- Higher Quality Content
- 60% Fewer Hallucinations
Browse by: Latest US Patents, China's latest patents, Technical Efficacy Thesaurus, Application Domain, Technology Topic, Popular Technical Reports.
© 2025 PatSnap. All rights reserved.Legal|Privacy policy|Modern Slavery Act Transparency Statement|Sitemap|About US| Contact US: help@patsnap.com