Thermal power plant condenser vacuum degree prediction method based on multi-layer LSTM
A technology for condensers and thermal power plants, applied in the field of prediction of vacuum degree of thermal power plant condensers based on multi-layer long-term short-term memory neural network, can solve the problem of poor generalization ability of the model, slow training speed, and failure to consider the condenser Vacuum time series and other issues
- Summary
- Abstract
- Description
- Claims
- Application Information
AI Technical Summary
Problems solved by technology
Method used
Image
Examples
Embodiment Construction
[0072] In order to make the purpose, technical solutions and advantages of the embodiments of the present invention more clear, the following will clearly and completely describe the technical solutions of the embodiments of the present invention in conjunction with the drawings of the embodiments of the present invention. Apparently, the described embodiments are some, not all, embodiments of the present invention. All other embodiments obtained by those skilled in the art based on the described embodiments of the present invention belong to the protection scope of the present invention.
[0073] In order to solve the problem that the prediction method of the vacuum degree of the condenser is difficult to dig deep into the data so that the prediction accuracy is not high, the present invention provides a method for predicting the vacuum degree of the condenser based on a multilayer long short-term memory network (Multilayer_LSTM), such as figure 1 As shown, the method include...
PUM
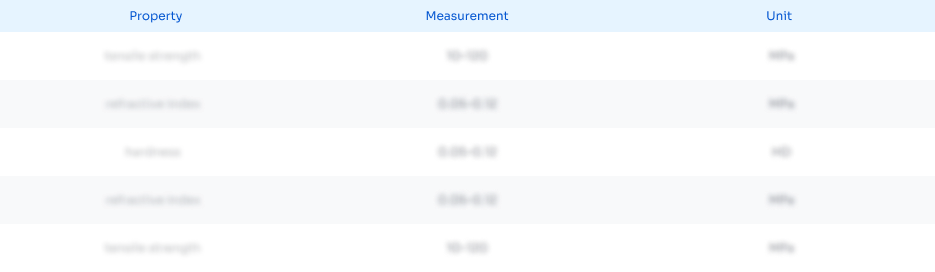
Abstract
Description
Claims
Application Information
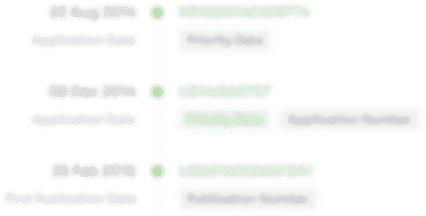
- R&D Engineer
- R&D Manager
- IP Professional
- Industry Leading Data Capabilities
- Powerful AI technology
- Patent DNA Extraction
Browse by: Latest US Patents, China's latest patents, Technical Efficacy Thesaurus, Application Domain, Technology Topic, Popular Technical Reports.
© 2024 PatSnap. All rights reserved.Legal|Privacy policy|Modern Slavery Act Transparency Statement|Sitemap|About US| Contact US: help@patsnap.com