HRRP target-free adversarial sample generation method based on deep learning
An adversarial sample, deep learning technology, applied in neural learning methods, biological neural network models, instruments, etc., can solve the problems of undiscovered, deep learning algorithm's radio signal classification vulnerable to attack, and destructive classifier classification performance. , to achieve the effect of high computational efficiency and improved security
- Summary
- Abstract
- Description
- Claims
- Application Information
AI Technical Summary
Problems solved by technology
Method used
Image
Examples
Embodiment
[0025] Aiming at the problem that the deep learning method is vulnerable to attack by adversarial samples, the present invention proposes a method for generating non-target attack adversarial samples for HRRP. Among them, the problem of selecting the coefficients of the disturbance in the FGSM algorithm is solved by the binary search method, and the general disturbance is generated by the aggregation method. Some basic concepts related to the present invention are:
[0026] 1. Deep neural network: Deep neural network refers to a multi-layer neural network, which is a technology in the field of machine learning. Its characteristic is that the input of the hidden layer node is the output of the upper layer network plus the bias, and each hidden layer node calculates its weighted input mean, and the output of the hidden layer node is the result of the nonlinear activation function. At the same time, multiple The benefit of layered neural networks is the ability to represent comp...
PUM
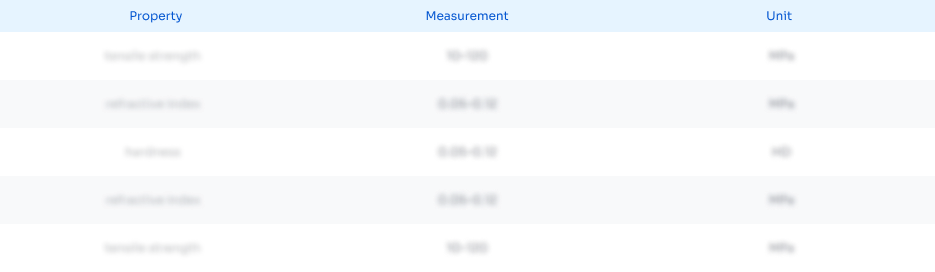
Abstract
Description
Claims
Application Information
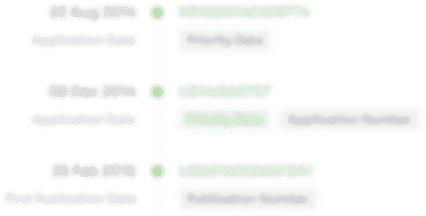
- R&D Engineer
- R&D Manager
- IP Professional
- Industry Leading Data Capabilities
- Powerful AI technology
- Patent DNA Extraction
Browse by: Latest US Patents, China's latest patents, Technical Efficacy Thesaurus, Application Domain, Technology Topic, Popular Technical Reports.
© 2024 PatSnap. All rights reserved.Legal|Privacy policy|Modern Slavery Act Transparency Statement|Sitemap|About US| Contact US: help@patsnap.com