Driving fatigue detection method and system combining pseudo 3D convolutional neural network and attention mechanism
A convolutional neural network and driving fatigue technology, applied in the field of intelligent video analysis, can solve problems such as large number of prediction model parameters, failure to remove recognition interference, and failure to consider spatial correlation, etc., to improve prediction performance and increase correlation , increasing the effect of global correlation
- Summary
- Abstract
- Description
- Claims
- Application Information
AI Technical Summary
Problems solved by technology
Method used
Image
Examples
Embodiment
[0057] A driving fatigue detection method combining a pseudo 3D convolutional neural network and an attention mechanism proposed by the present invention comprises the following steps:
[0058] Step 1, extracting and processing the video frame sequence from the driving video;
[0059] Step 2, using the pseudo 3D convolution module for spatio-temporal feature learning;
[0060] Step 3, build the P3D-Attention module, and use the attention mechanism to pay attention to the channel and feature map;
[0061] Step 4, use 2D global average pooling layer instead of 3D global average pooling layer to obtain more expressive features, and use softmax classification layer for classification.
[0062] In the step 1, the method of extracting and processing the video frame sequence from the video is as follows: the video is collected for about 5 seconds each time, and the number of video frames extracted each time is 180.
[0063] The pseudo-3D convolution module for spatio-temporal featu...
PUM
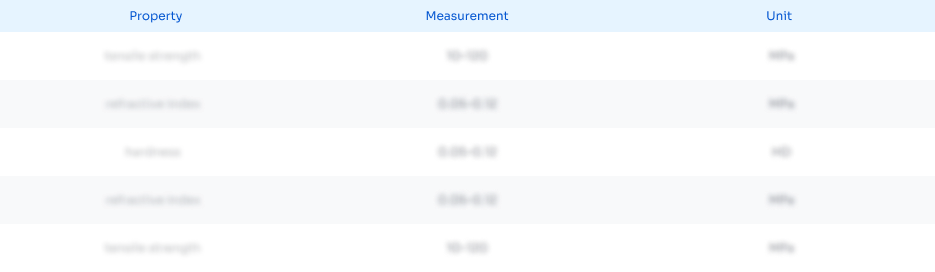
Abstract
Description
Claims
Application Information
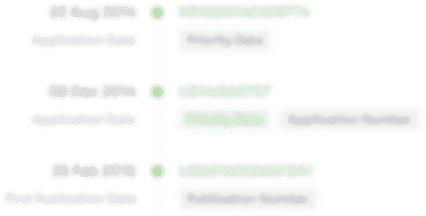
- R&D
- Intellectual Property
- Life Sciences
- Materials
- Tech Scout
- Unparalleled Data Quality
- Higher Quality Content
- 60% Fewer Hallucinations
Browse by: Latest US Patents, China's latest patents, Technical Efficacy Thesaurus, Application Domain, Technology Topic, Popular Technical Reports.
© 2025 PatSnap. All rights reserved.Legal|Privacy policy|Modern Slavery Act Transparency Statement|Sitemap|About US| Contact US: help@patsnap.com