Improved non-convex robust principal component analysis method
A principal component analysis, non-convex technology, applied in the direction of instruments, character and pattern recognition, computer parts, etc., can solve problems such as hindering development, loss of information in principal component analysis methods, and difficulty in solving problems, and achieves improved effects and richness. The effect of structured information
- Summary
- Abstract
- Description
- Claims
- Application Information
AI Technical Summary
Problems solved by technology
Method used
Image
Examples
Embodiment Construction
[0041] In order to make the purpose, features and advantages of the present invention more obvious and understandable, the technical solutions in the embodiments of the present invention will be clearly and completely described below in conjunction with the accompanying drawings in the embodiments of the present invention. Obviously, the following The described embodiments are only some, but not all, embodiments of the present invention. Based on the embodiments of the present invention, all other embodiments obtained by persons of ordinary skill in the art without making creative efforts belong to the protection scope of the present invention.
[0042] The technical solutions of the present invention will be further described below in conjunction with the accompanying drawings and through specific implementation methods.
[0043] like figure 1As shown in the figure, the figure is a structural block diagram of an improved non-convex robust principal component analysis method....
PUM
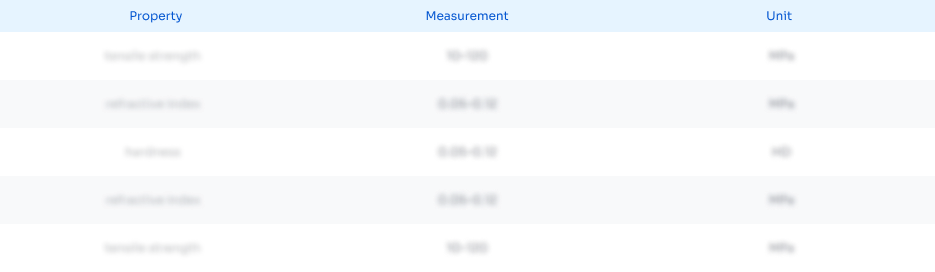
Abstract
Description
Claims
Application Information
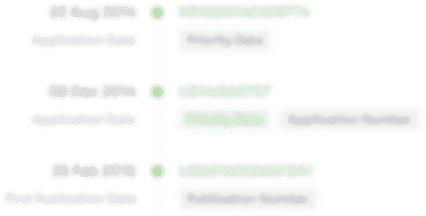
- Generate Ideas
- Intellectual Property
- Life Sciences
- Materials
- Tech Scout
- Unparalleled Data Quality
- Higher Quality Content
- 60% Fewer Hallucinations
Browse by: Latest US Patents, China's latest patents, Technical Efficacy Thesaurus, Application Domain, Technology Topic, Popular Technical Reports.
© 2025 PatSnap. All rights reserved.Legal|Privacy policy|Modern Slavery Act Transparency Statement|Sitemap|About US| Contact US: help@patsnap.com