Cross-modal hash retrieval method based on self-learning
A cross-modal, self-learning technology, applied in the computer field, can solve problems such as ignoring binary constraints, errors, and reducing the ability to distinguish binary codes, so as to reduce coding errors and improve quality
- Summary
- Abstract
- Description
- Claims
- Application Information
AI Technical Summary
Problems solved by technology
Method used
Image
Examples
Embodiment Construction
[0019] Embodiments of the present invention will be further described below in conjunction with the accompanying drawings.
[0020] figure 1 A framework diagram of a self-learning based cross-modal hash retrieval method. For simplicity of description, the present invention uses the two most common modalities (text and image) as samples for cross-modal retrieval. First, the co-matrix factorization technique is employed to project the feature data of different modalities into a common latent semantic space. Secondly, the common semantic space is rotated to minimize the variance of data of different dimensions through orthogonal transformation technology, so that the binary quantization loss is minimized, so that samples of the same category but not spatially related can be further converted into similar binary codes. Also, consider maintaining intra-modal and inter-modal similarities. For intra-modal similarity, local geometry is used for learning; for inter-modal similarity,...
PUM
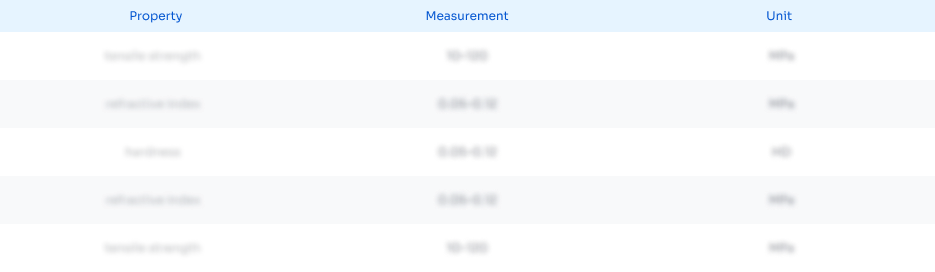
Abstract
Description
Claims
Application Information
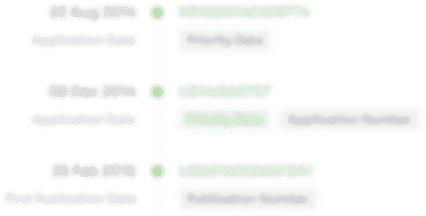
- R&D Engineer
- R&D Manager
- IP Professional
- Industry Leading Data Capabilities
- Powerful AI technology
- Patent DNA Extraction
Browse by: Latest US Patents, China's latest patents, Technical Efficacy Thesaurus, Application Domain, Technology Topic, Popular Technical Reports.
© 2024 PatSnap. All rights reserved.Legal|Privacy policy|Modern Slavery Act Transparency Statement|Sitemap|About US| Contact US: help@patsnap.com