Improved deep reinforcement learning method and system based on Double DQN
A reinforcement learning and deep technology, applied in the field of reinforcement learning, can solve problems such as inability to converge
- Summary
- Abstract
- Description
- Claims
- Application Information
AI Technical Summary
Problems solved by technology
Method used
Image
Examples
Embodiment Construction
[0059] In order to make the purpose, technical solution and advantages of the present application clearer, the present application will be further described in detail below in conjunction with the accompanying drawings and embodiments. It should be understood that the specific embodiments described here are only used to explain the present application, and are not intended to limit the present application.
[0060] In one embodiment, combined with figure 1 , provides an improved deep reinforcement learning method based on Double Deep Q-Learning Network, which includes the following steps:
[0061] Step 1, initialize the environment and DQN network parameters;
[0062] Here, the environment includes: state space action space and reward function r; DQN network parameters include current value neural network parameters, target value neural network parameters, DQN error function and playback memory unit Among them, the neural network parameters include the number of network...
PUM
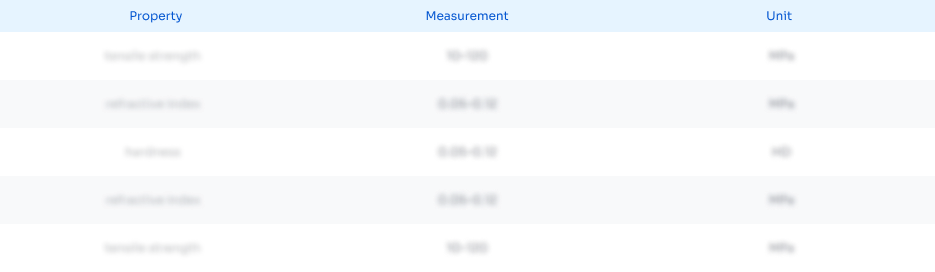
Abstract
Description
Claims
Application Information
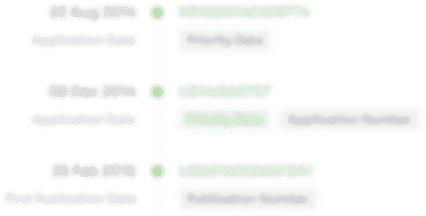
- R&D Engineer
- R&D Manager
- IP Professional
- Industry Leading Data Capabilities
- Powerful AI technology
- Patent DNA Extraction
Browse by: Latest US Patents, China's latest patents, Technical Efficacy Thesaurus, Application Domain, Technology Topic, Popular Technical Reports.
© 2024 PatSnap. All rights reserved.Legal|Privacy policy|Modern Slavery Act Transparency Statement|Sitemap|About US| Contact US: help@patsnap.com