A weakly supervised fine-grained image recognition method based on visual self-attention mechanism
An image recognition and attention technology, applied in the field of computer vision, can solve problems such as local optimal solutions and complex training methods, and achieve the effects of reducing computational costs, enhancing robustness, and reducing design burdens
- Summary
- Abstract
- Description
- Claims
- Application Information
AI Technical Summary
Problems solved by technology
Method used
Image
Examples
Embodiment Construction
[0044] A weakly supervised fine-grained image recognition method based on a visual self-attention mechanism, comprising the following steps:
[0045] Step 1: In the preprocessing stage, the original image of any size is scaled to 600×600 pixels, and on this basis, a 448×448 pixel area is cut out with the center of the image as the origin, according to the mean [0.485, 0.456, 0.406] and standard deviation [0.229, 0.224, 0.225] Normalize the clipping area, and then input the normalized image into the fine-grained recognition model based on the visual self-attention mechanism;
[0046] Step 2: The input image outputs a 14×14×2048-dimensional feature tensor through a shared convolutional neural network. The student-model uses the anchor frame idea of the region proposal network RPN commonly used in the field of target detection to set the step size to 1, 2, 2, Three 3×3 convolutional layers with 128 output channels are sequentially connected to the shared base network to reduce ...
PUM
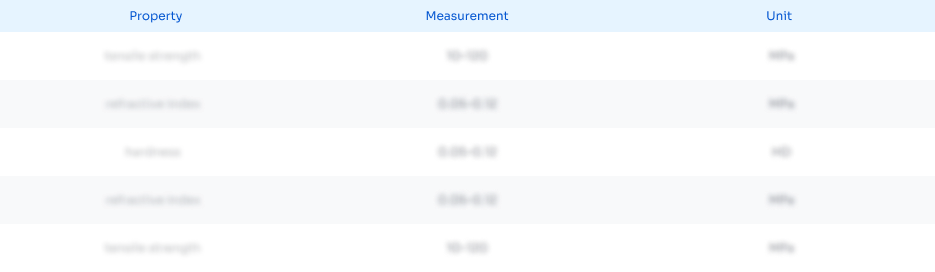
Abstract
Description
Claims
Application Information
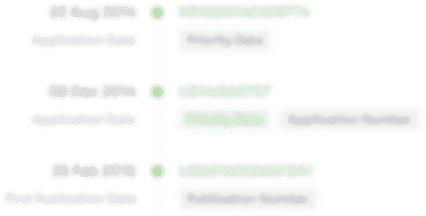
- R&D
- Intellectual Property
- Life Sciences
- Materials
- Tech Scout
- Unparalleled Data Quality
- Higher Quality Content
- 60% Fewer Hallucinations
Browse by: Latest US Patents, China's latest patents, Technical Efficacy Thesaurus, Application Domain, Technology Topic, Popular Technical Reports.
© 2025 PatSnap. All rights reserved.Legal|Privacy policy|Modern Slavery Act Transparency Statement|Sitemap|About US| Contact US: help@patsnap.com