Dimensionality reduction and reconstruction method of spatial multi-dimensional wind power data based on rbf kernel function
A wind power and data dimensionality reduction technology, which is applied in the fields of instrumentation, calculation, character and pattern recognition, etc., can solve the problems of pre-image reconstruction of dimensionality reduction results, difficult data essential features, and inability to extract nonlinear characteristics of wind power data.
- Summary
- Abstract
- Description
- Claims
- Application Information
AI Technical Summary
Problems solved by technology
Method used
Image
Examples
Embodiment Construction
[0071] Such as figure 1 As shown, the spatial multi-dimensional wind power data dimensionality reduction and reconstruction method ORBF-KPCA based on RBF kernel function includes the following steps:
[0072] Step 1: Collect N hour-level wind power measured samples of m wind farms in a certain area X N×m =[x 1 , x 2 ,...,x N ] T , where x 1 , x 2 ,...,x N Represents the m-dimensional wind power sample vector corresponding to N observation times.
[0073] Step 2: If figure 2 As shown, based on N hour-level wind power measured samples of m wind farms in a certain area, the KPCA method is used to obtain the dimensionality reduction results of spatial multi-dimensional wind power.
[0074] Step 2.1: Input the data matrix X of n power observation samples of m wind farms N×m =[x 1 , x 2 ,...,x N ] T , where x 1 , x 2 ,...,x N Indicates the m-dimensional wind power sample vector corresponding to N observation times, and calculates the RBF kernel matrix K=[k ij ] N...
PUM
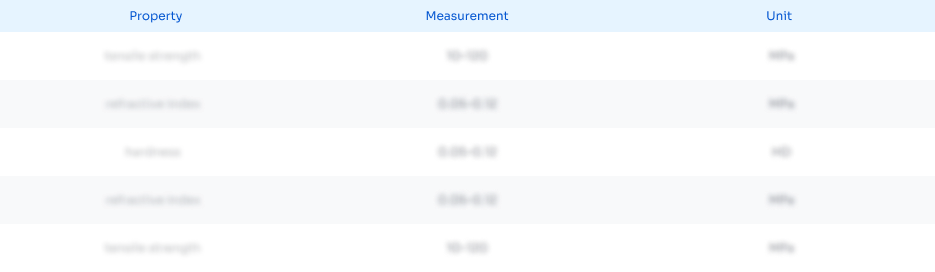
Abstract
Description
Claims
Application Information
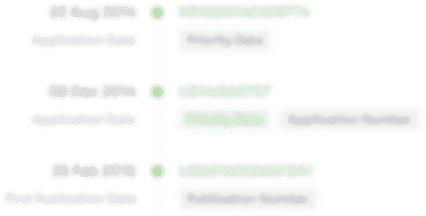
- R&D Engineer
- R&D Manager
- IP Professional
- Industry Leading Data Capabilities
- Powerful AI technology
- Patent DNA Extraction
Browse by: Latest US Patents, China's latest patents, Technical Efficacy Thesaurus, Application Domain, Technology Topic, Popular Technical Reports.
© 2024 PatSnap. All rights reserved.Legal|Privacy policy|Modern Slavery Act Transparency Statement|Sitemap|About US| Contact US: help@patsnap.com