Lithium battery capacity online estimation method based on convolutional neural network
A convolutional neural network, lithium battery technology, applied in the field of fault prediction and health management, can solve problems such as difficult to achieve
- Summary
- Abstract
- Description
- Claims
- Application Information
AI Technical Summary
Problems solved by technology
Method used
Image
Examples
Embodiment Construction
[0020] The method for online estimation of lithium battery capacity proposed by the present invention will be further described below in conjunction with the description of the drawings and specific implementation examples.
[0021] Such as figure 1 As shown, an online estimation method of lithium battery capacity based on convolutional neural network includes the following steps:
[0022] S1. In this embodiment, four lithium battery charging and discharging experimental data provided by the Center for Advanced Life Cycle Engineering (CALCE) of the University of Maryland, respectively CS2-35, CS2-36, CS2-37, and CS2-38, are used as data sources , where CS2-36, CS2-37, and CS2-38 batteries are used as reference batteries, and CS2-35 is used as the battery to be tested. In this experiment, four batteries with a design capacity of 1.1Ah were subjected to the same standard charging process at room temperature with a constant current rate of 0.5C until the voltage reached 4.2V, an...
PUM
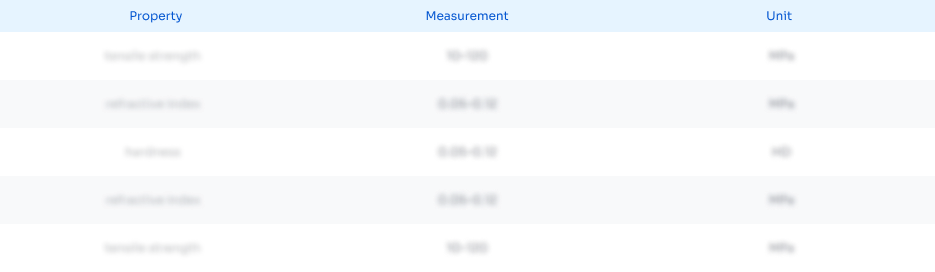
Abstract
Description
Claims
Application Information
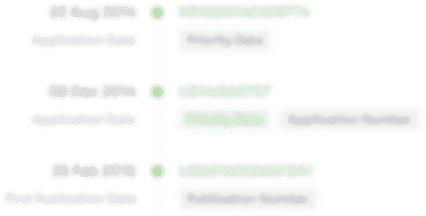
- R&D Engineer
- R&D Manager
- IP Professional
- Industry Leading Data Capabilities
- Powerful AI technology
- Patent DNA Extraction
Browse by: Latest US Patents, China's latest patents, Technical Efficacy Thesaurus, Application Domain, Technology Topic, Popular Technical Reports.
© 2024 PatSnap. All rights reserved.Legal|Privacy policy|Modern Slavery Act Transparency Statement|Sitemap|About US| Contact US: help@patsnap.com