Training method and training system for non-autoregressive machine translation model based on task-level curriculum learning
A technology for machine translation and training methods, applied in natural language translation, computational models, machine learning, etc., can solve the problem of low accuracy of non-autoregressive machine translation models, and achieve improved inference efficiency, accuracy, and acceleration. Effect
- Summary
- Abstract
- Description
- Claims
- Application Information
AI Technical Summary
Problems solved by technology
Method used
Image
Examples
Embodiment
[0091] This implementation uses four standard translation datasets: IWSLT14 German-English (De-En) dataset, IWSLT16 English-German (En-De) dataset and WMT14 English-German (En-De) dataset. This implementation reverses the WMT14 English-German dataset to obtain a fourth dataset, the WMT14 German-English (De-En) dataset. Among them, the IWSLT14 German-English (De-En) dataset and the IWSLT16 English-German (En-De) dataset are from the literature M.Cettolo, C.Girardi, and M.Federico.2012.WIT3:Web Inventory of Transcribed and Translated Talks .InProc.of EAMT, pp.261-268, Trento, Italy; WMT14 English-German (En-De) dataset from the ACL Ninth Workshop on Statistical Machine Translation (https: / / www.statmt.org / wmt14 / translation-task.html)
[0092] Among them, the number of bilingual sentence pairs used for training, development, and testing in the IWSLT14 dataset is 153k, 7k, and 7k, respectively. The number of bilingual sentence pairs used by IWSLT16 for training, development, and...
PUM
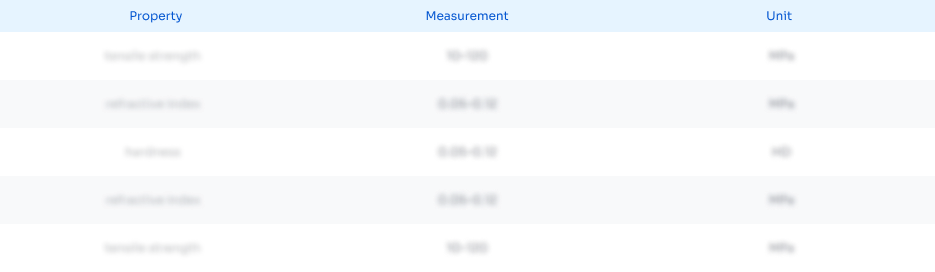
Abstract
Description
Claims
Application Information
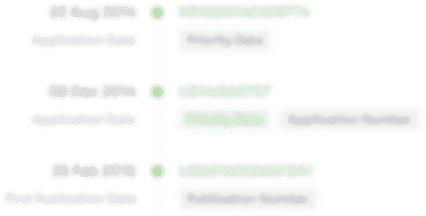
- R&D Engineer
- R&D Manager
- IP Professional
- Industry Leading Data Capabilities
- Powerful AI technology
- Patent DNA Extraction
Browse by: Latest US Patents, China's latest patents, Technical Efficacy Thesaurus, Application Domain, Technology Topic, Popular Technical Reports.
© 2024 PatSnap. All rights reserved.Legal|Privacy policy|Modern Slavery Act Transparency Statement|Sitemap|About US| Contact US: help@patsnap.com