Adversarial sample defense method and system based on VAE-GAN
A VAE-GAN, adversarial sample technology, applied in the field of adversarial sample defense methods and systems based on VAE-GAN, can solve the problems of the decline of the original classifier classification accuracy, high defense training costs, and poor generality of defense solutions, and achieve defense effects. Good, low training cost, fast preprocessing
- Summary
- Abstract
- Description
- Claims
- Application Information
AI Technical Summary
Problems solved by technology
Method used
Image
Examples
Embodiment Construction
[0050] In order to make the objectives, technical solutions and advantages of the present invention clearer, the present invention will be further described in detail below in conjunction with embodiments. It should be understood that the specific embodiments described herein are only used to explain the present invention, but not to limit the present invention.
[0051] The traditional denoising of images and reduction of over-fitting of deep neural networks can no longer defend against the attacks of these adversarial samples, and the current defense schemes have the disadvantages of high training costs and poor defensive migration capabilities.
[0052] In the current defense scheme, input preprocessing will cause the quality of the input image to decrease and reduce the classification accuracy of the original noise-free image. At the same time, most of the defense schemes have a better defense effect on adversarial samples with larger disturbances. The smaller the disturbance, t...
PUM
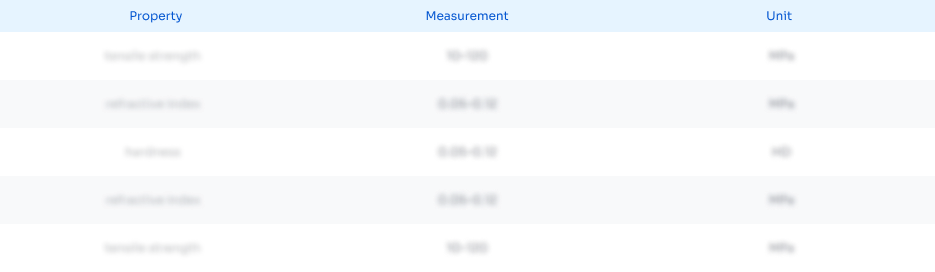
Abstract
Description
Claims
Application Information
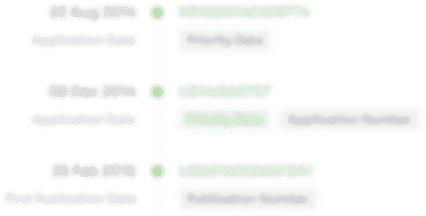
- R&D Engineer
- R&D Manager
- IP Professional
- Industry Leading Data Capabilities
- Powerful AI technology
- Patent DNA Extraction
Browse by: Latest US Patents, China's latest patents, Technical Efficacy Thesaurus, Application Domain, Technology Topic, Popular Technical Reports.
© 2024 PatSnap. All rights reserved.Legal|Privacy policy|Modern Slavery Act Transparency Statement|Sitemap|About US| Contact US: help@patsnap.com