Rolling bearing fault diagnosis method for improving model migration strategy
A rolling bearing and fault diagnosis technology, which is applied in neural learning methods, biological neural network models, character and pattern recognition, etc., can solve the problems of difficult comprehensive acquisition of rolling bearing vibration data, large differences in data distribution in the same state, and low recognition accuracy. Achieve the effect of avoiding the phenomenon of gradient non-convergence, avoiding the phenomenon of gradient non-convergence, and improving the strategy
- Summary
- Abstract
- Description
- Claims
- Application Information
AI Technical Summary
Problems solved by technology
Method used
Image
Examples
Embodiment Construction
[0050] In conjunction with the accompanying drawings, the implementation process of the rolling bearing fault diagnosis method of the improved model migration strategy described in the present invention is described in detail as follows:
[0051] 1 yuan learning
[0052] The related theory of meta-learning algorithm and its application in the field of supervised learning is expounded.
[0053] 1.1 Meta-Learning Theory
[0054] Meta-learning, or learning to learn, is the discipline of systematically looking at how other learning methods perform on a broad range of learning tasks, learning from acquired experience or metadata, to learn new tasks faster relative to other learning methods [28] . Meta-learning first appeared in cognitive psychology and is becoming a formal concept in machine learning in recent years.
[0055] Meta-learning pays more attention to how to improve the learning ability of neural networks, and is widely used in fields such as enhancing memory, predict...
PUM
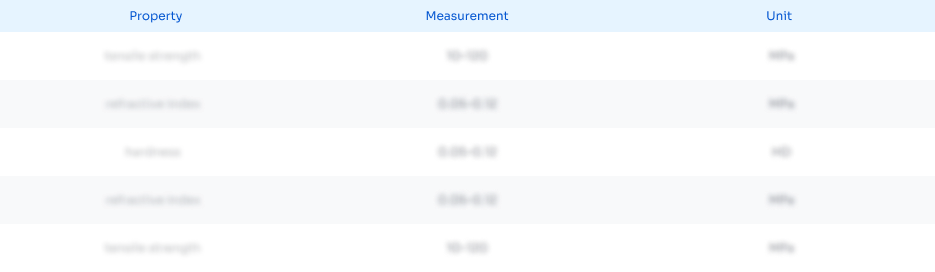
Abstract
Description
Claims
Application Information
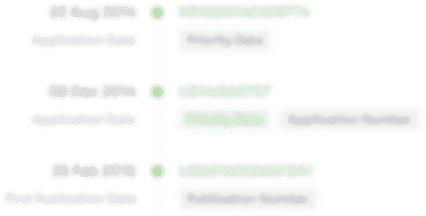
- R&D
- Intellectual Property
- Life Sciences
- Materials
- Tech Scout
- Unparalleled Data Quality
- Higher Quality Content
- 60% Fewer Hallucinations
Browse by: Latest US Patents, China's latest patents, Technical Efficacy Thesaurus, Application Domain, Technology Topic, Popular Technical Reports.
© 2025 PatSnap. All rights reserved.Legal|Privacy policy|Modern Slavery Act Transparency Statement|Sitemap|About US| Contact US: help@patsnap.com