Neural network model construction method based on pruning and related device
A technology of neural network model and construction method, applied in biological neural network model, neural learning method, neural architecture, etc., can solve problems such as limited applicability, large loss of model accuracy, and difficulty in designing compression optimization methods.
- Summary
- Abstract
- Description
- Claims
- Application Information
AI Technical Summary
Problems solved by technology
Method used
Image
Examples
Embodiment 1
[0052] For ease of understanding, see figure 1 , Embodiment 1 of a pruning-based neural network model construction method provided by the present application, including:
[0053] Step 101. Arrange the initial weight values of the preset neural network models in a preset order to obtain an initial weight value sequence.
[0054] It should be noted that the preset neural network model is a trained neural network model that can be directly applied to actual object recognition, positioning and other scenarios. The weight value of the preset neural network model prepared at this time is defined as the initial weight value, and each initial weight value corresponds to a branch of the preset neural network model. The meaning of pruning is to reduce the number of weight values in the model. The most direct way is to set some weight values to zero; arrange the weight values in a regular order, either in ascending or descending order, and the initial weight value arrangement of...
Embodiment 2
[0066] For ease of understanding, see figure 2 , the present application provides a second embodiment of a pruning-based neural network model construction method, including:
[0067] Step 201, performing pre-training operation on the original neural network model to obtain a preset neural network model.
[0068] It should be noted that the specific method of the pre-training operation is mainly for the specific application object of the neural network model. Through the pre-training operation, the model can learn and memorize the feature points in the specific task, clarify the purpose of the task, etc., and obtain the preset neural network model. The network model can directly perform corresponding tasks. For example, using a neural network model for image recognition, first use a large number of images to pre-train the neural network to obtain a preset neural network model with a certain accuracy, and then use a small amount of test data set, Or input the image of the targ...
PUM
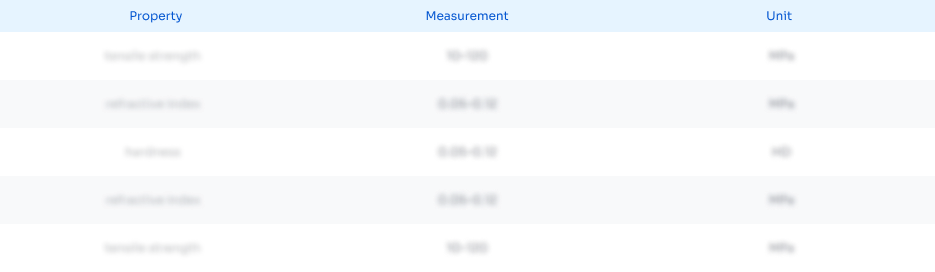
Abstract
Description
Claims
Application Information
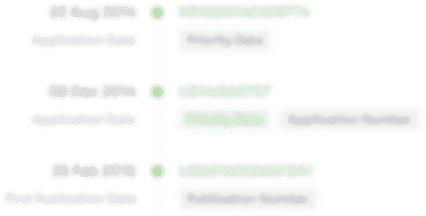
- Generate Ideas
- Intellectual Property
- Life Sciences
- Materials
- Tech Scout
- Unparalleled Data Quality
- Higher Quality Content
- 60% Fewer Hallucinations
Browse by: Latest US Patents, China's latest patents, Technical Efficacy Thesaurus, Application Domain, Technology Topic, Popular Technical Reports.
© 2025 PatSnap. All rights reserved.Legal|Privacy policy|Modern Slavery Act Transparency Statement|Sitemap|About US| Contact US: help@patsnap.com