Rail transit passenger flow prediction method based on dynamic hypergraph convolution network
A convolutional network and rail transit technology, applied in the field of graph theory and deep learning, to achieve the effect of improving prediction accuracy and improving accuracy
- Summary
- Abstract
- Description
- Claims
- Application Information
AI Technical Summary
Problems solved by technology
Method used
Image
Examples
Embodiment Construction
[0035] Rail traffic flow prediction is a fundamental problem in the field of smart transportation. The dynamic hypergraph convolution network proposed by the method of the present invention can model the topological structure and passenger flow characteristics of rail transit, and realize the improvement of prediction accuracy. We actually used the historical data of rail transit passenger flow in Beijing in July, September, and December of 2015 for a total of three months to train the dynamic hypergraph convolutional network and test the accuracy of the prediction results.
[0036]The input of the dynamic hypergraph convolutional network is the traffic flow data represented by the subway passenger flow and the traffic network structure represented by the subway line structure. The traffic flow data is analyzed by OD, and the travel rules of large passenger flow are obtained through clustering algorithm, and the hyperedge is implied by this. Taking the morning peak period in ...
PUM
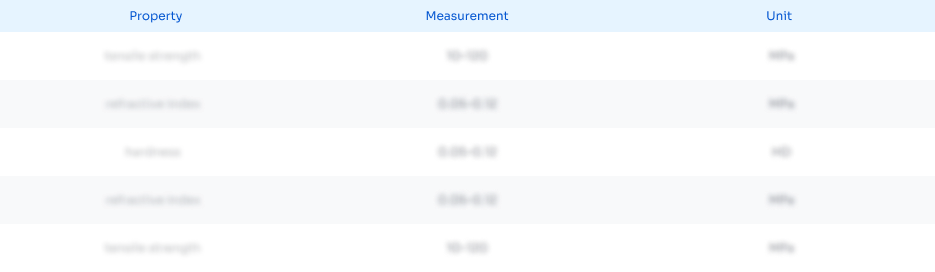
Abstract
Description
Claims
Application Information
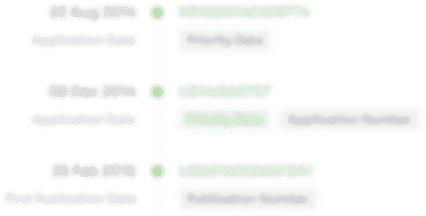
- R&D Engineer
- R&D Manager
- IP Professional
- Industry Leading Data Capabilities
- Powerful AI technology
- Patent DNA Extraction
Browse by: Latest US Patents, China's latest patents, Technical Efficacy Thesaurus, Application Domain, Technology Topic, Popular Technical Reports.
© 2024 PatSnap. All rights reserved.Legal|Privacy policy|Modern Slavery Act Transparency Statement|Sitemap|About US| Contact US: help@patsnap.com