Convolutional neural network training method, electroencephalogram signal recognition method and device and medium
A convolutional neural network and electroencephalographic signal technology, applied in the field of convolutional neural network training method, electroencephalographic signal recognition method, device and medium, can solve the problem of unfavorable application of brain-computer interface, electroencephalographic signal noise interference, electroencephalic signal Identify problems such as unfavorableness, and achieve the effect of reducing over-fitting, improving the recognition effect, and increasing the amount of training data.
- Summary
- Abstract
- Description
- Claims
- Application Information
AI Technical Summary
Problems solved by technology
Method used
Image
Examples
Embodiment Construction
[0046] In this example, refer to figure 1 , the convolutional neural network training method includes the following steps:
[0047] P1. Execute multiple acquisition processes; each acquisition process is used to acquire EEG signals;
[0048] P2. Perform time-domain data enhancement and frequency-domain data enhancement on EEG signals;
[0049] P3. Training a convolutional neural network using EEG signals augmented with time-domain data and frequency-domain data.
[0050] In this embodiment, when performing each acquisition process in step P1, the subject is required to imagine a certain type of action, so that the subject's brain generates EEG signals, which pass through the brain including C3, Cz and C4 channels. The electrical signal acquisition instrument acquires the electroencephalogram signal. In this embodiment, the subject may be required to imagine the same type of action in each acquisition process, which can reduce interference caused by different types of imagin...
PUM
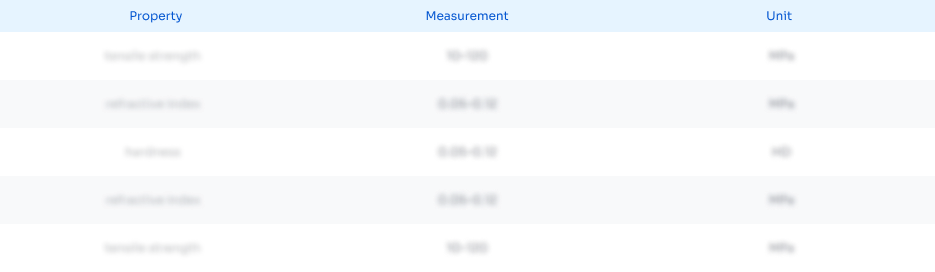
Abstract
Description
Claims
Application Information
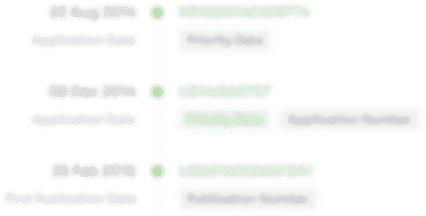
- R&D Engineer
- R&D Manager
- IP Professional
- Industry Leading Data Capabilities
- Powerful AI technology
- Patent DNA Extraction
Browse by: Latest US Patents, China's latest patents, Technical Efficacy Thesaurus, Application Domain, Technology Topic, Popular Technical Reports.
© 2024 PatSnap. All rights reserved.Legal|Privacy policy|Modern Slavery Act Transparency Statement|Sitemap|About US| Contact US: help@patsnap.com