Medical image segmentation method based on 3D dynamic edge insensitivity loss function
A loss function and medical image technology, applied in the field of medical image processing, can solve the problems affecting the generalization of the segmentation model and the high risk of model overfitting, so as to improve the generalization performance, improve the generalization and accuracy, and reduce the network The effect of the training parameters
- Summary
- Abstract
- Description
- Claims
- Application Information
AI Technical Summary
Problems solved by technology
Method used
Image
Examples
Embodiment 1
[0043] Embodiment 1 Using the method of the present invention to perform multi-region segmentation of the prostate
[0044] The medical image segmentation method based on the 3D dynamic edge insensitivity loss function provided by the present invention is end-to-end, and the specific implementation process of the embodiment is as follows:
[0045] Step 1. There are 68 cases in the training set data, which come from the ISBI data set. First, intensity normalization and histogram equalization are performed on the image, and the entire image is divided into many small blocks of pixels for nonlinear stretching, so that the local gray histogram is evenly distributed. In order for the network to correctly learn the spatial semantics, all MR voxels are resampled to a uniform size using third-order spline interpolation. Nearest neighbor interpolation is used for the corresponding segmentation annotations. Random transformations mainly including random rotation, shearing, scaling and...
PUM
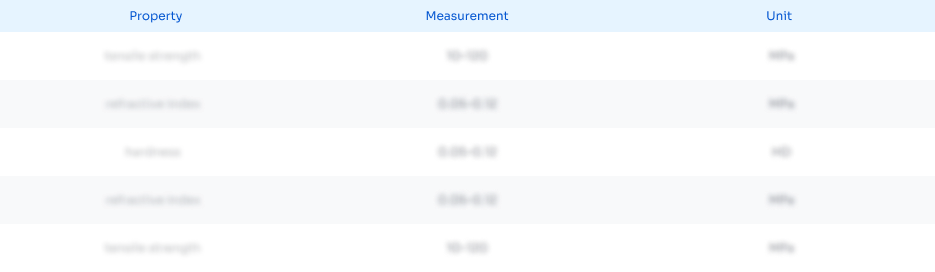
Abstract
Description
Claims
Application Information
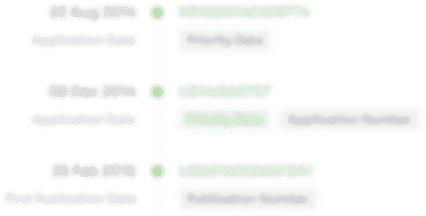
- R&D
- Intellectual Property
- Life Sciences
- Materials
- Tech Scout
- Unparalleled Data Quality
- Higher Quality Content
- 60% Fewer Hallucinations
Browse by: Latest US Patents, China's latest patents, Technical Efficacy Thesaurus, Application Domain, Technology Topic, Popular Technical Reports.
© 2025 PatSnap. All rights reserved.Legal|Privacy policy|Modern Slavery Act Transparency Statement|Sitemap|About US| Contact US: help@patsnap.com