Remote sensing image segmentation algorithm based on convolutional neural network
A technology of convolutional neural network and segmentation algorithm, which is applied in the field of semantic segmentation algorithm, can solve the problems of slow manual analysis and insufficient processing of remote sensing image information, so as to improve the speed, capture rate of effective information, improve segmentation accuracy, and clear The effect of the scene analysis effect
- Summary
- Abstract
- Description
- Claims
- Application Information
AI Technical Summary
Problems solved by technology
Method used
Image
Examples
Embodiment Construction
[0039] The present invention will be further described in detail below in conjunction with the accompanying drawings and embodiments.
[0040] The invention uses a convolutional neural network to perform semantic segmentation on remote sensing images, that is, performs pixel-level classification and pixel coloring on different types of objects to achieve the purpose of semantic segmentation. What the present invention aims to achieve is the category distinction of each pixel, and the deep residual network structure is combined with the pyramid pooling model to realize the semantic segmentation task of the remote sensing image by the deep neural network.
[0041] In the traditional sense, the depth of the network has a great influence on the final classification and recognition of images, so the conventional idea is that the deeper the network design, the better, but many experimental results have proved that when the network stack is very deep, The effect will be worse. Analy...
PUM
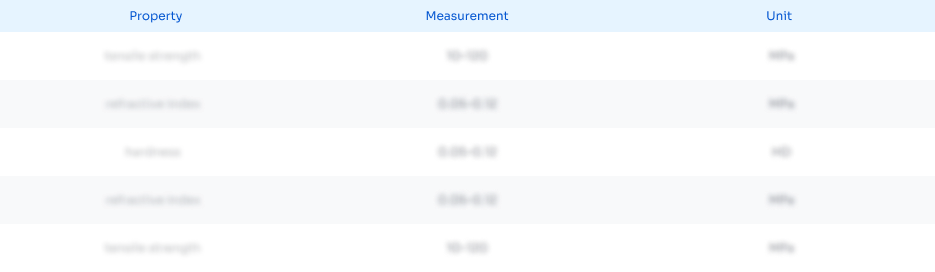
Abstract
Description
Claims
Application Information
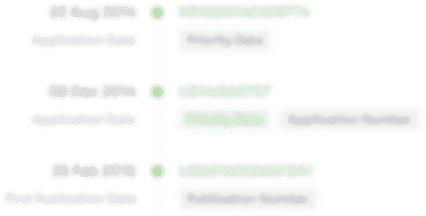
- R&D Engineer
- R&D Manager
- IP Professional
- Industry Leading Data Capabilities
- Powerful AI technology
- Patent DNA Extraction
Browse by: Latest US Patents, China's latest patents, Technical Efficacy Thesaurus, Application Domain, Technology Topic, Popular Technical Reports.
© 2024 PatSnap. All rights reserved.Legal|Privacy policy|Modern Slavery Act Transparency Statement|Sitemap|About US| Contact US: help@patsnap.com