A meta-knowledge fine-tuning method and platform for multi-task language models
A language model and multi-task technology, applied in the direction of reasoning methods, semantic analysis, unstructured text data retrieval, etc., can solve problems such as the limited effect of compression models, improve parameter initialization capabilities and generalization capabilities, and improve fine-tuning effects , Improve the effect of compression efficiency
- Summary
- Abstract
- Description
- Claims
- Application Information
AI Technical Summary
Problems solved by technology
Method used
Image
Examples
Embodiment Construction
[0029] Such as figure 1 As shown, the present invention is a multi-task language model-oriented meta-knowledge fine-tuning method and platform. On the downstream task multi-domain data set of the pre-trained language model, based on cross-domain typical score learning, the meta-knowledge of typical scores is used. -Knowledge fine-tunes downstream task scenarios, making it easier for meta-learners to fine-tune to any domain. The learned knowledge is highly generalizable and transferable, rather than limited to a specific domain. The resulting compression model The effect is suitable for data scenarios in different domains of the same task.
[0030] A meta-knowledge fine-tuning method oriented to a multi-task language model of the present invention, specifically comprising the following steps:
[0031] Step 1: Calculate the class prototypes of cross-domain data sets for similar tasks: Considering that multi-domain class prototypes can summarize the key semantic features of the ...
PUM
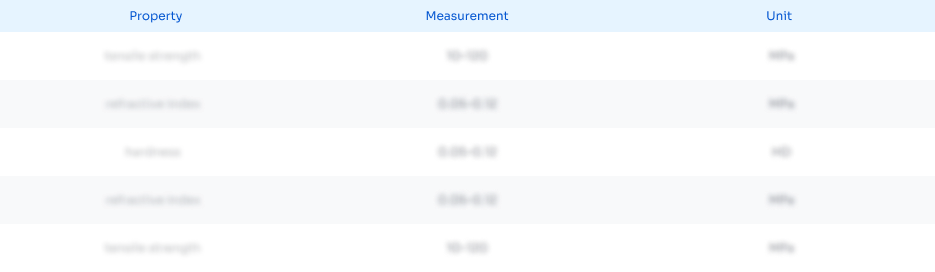
Abstract
Description
Claims
Application Information
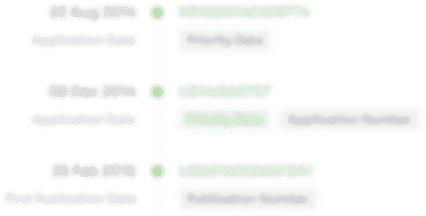
- R&D Engineer
- R&D Manager
- IP Professional
- Industry Leading Data Capabilities
- Powerful AI technology
- Patent DNA Extraction
Browse by: Latest US Patents, China's latest patents, Technical Efficacy Thesaurus, Application Domain, Technology Topic, Popular Technical Reports.
© 2024 PatSnap. All rights reserved.Legal|Privacy policy|Modern Slavery Act Transparency Statement|Sitemap|About US| Contact US: help@patsnap.com