Alarm root cause identification method based on causal network mining and graph attention network
A technology of causal network and identification method, which is applied in the direction of data exchange network, neural learning method, biological neural network model, etc., can solve the problems of complex fault location, failure to achieve results, error-prone and other problems, so as to save manpower, material and financial resources, improve The effect of predictive accuracy
- Summary
- Abstract
- Description
- Claims
- Application Information
AI Technical Summary
Problems solved by technology
Method used
Image
Examples
Embodiment Construction
[0029] The present invention will be described in further detail below in conjunction with the accompanying drawings and embodiments.
[0030] refer to figure 1 , in the Huawei wireless field, the occurrence of a fault will cause a large number of alarms to be generated. What needs to be done is to identify the root cause alarm (RA) from the real-time alarm information flow, and finally determine the root cause of the fault (RC). After many investigations, we found that the current monitoring and management of network alarms in Huawei's wireless field is mainly done manually. The specific problems are as follows: First, the process of fault demarcation is time-consuming and labor-intensive. The operation and maintenance cost is too high, and it cannot meet the current demand when a large number of fault alarms are concurrently issued. The second is that the efficiency of locating faults is low, which often leads to repeated or unnecessary on-site visits, which increases unnec...
PUM
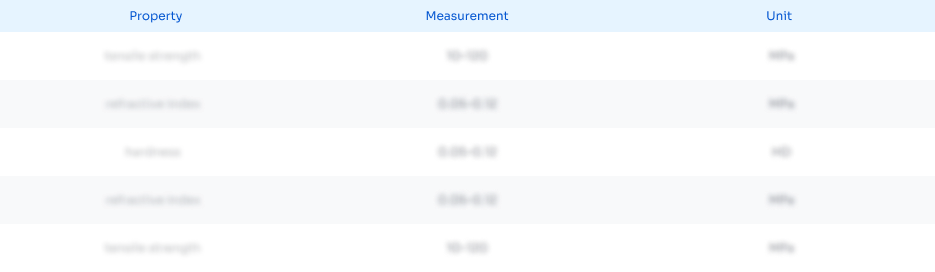
Abstract
Description
Claims
Application Information
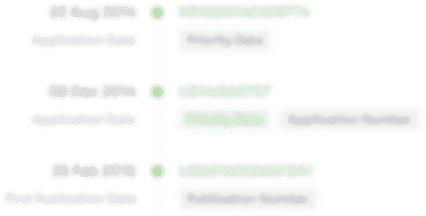
- R&D Engineer
- R&D Manager
- IP Professional
- Industry Leading Data Capabilities
- Powerful AI technology
- Patent DNA Extraction
Browse by: Latest US Patents, China's latest patents, Technical Efficacy Thesaurus, Application Domain, Technology Topic, Popular Technical Reports.
© 2024 PatSnap. All rights reserved.Legal|Privacy policy|Modern Slavery Act Transparency Statement|Sitemap|About US| Contact US: help@patsnap.com