Debris flow early warning method and system based on mechanism and machine learning coupling
A machine learning and early warning system technology, applied to instruments, alarms, climate sustainability, etc., can solve problems such as not taking into account the mechanism and process of debris flow, so as to improve forecast accuracy, comprehensive and accurate forecast results, and reduce false alarm rate Effect
- Summary
- Abstract
- Description
- Claims
- Application Information
AI Technical Summary
Problems solved by technology
Method used
Image
Examples
Embodiment 1
[0031] Such as figure 2 As shown, a debris flow early warning system based on the coupling of mechanism and machine learning includes a eigenvector set unit, a data input unit, and a model training unit; the eigenvalue parameters of the eigenvector set unit include historical precipitation values, forecast precipitation values, lower Data value of backing surface, risk level value of debris flow based on small watershed unit P i , i is the total number of small watershed units in the region;
[0032] The data input unit is used to input the feature vector set unit into the machine learning model;
[0033]The model training unit is used to train the machine learning model by calling the function interface in the machine learning library sklearn according to the data characteristics of the feature vector set, and perform machine learning model testing and parameter tuning.
[0034] In implementation, the machine learning model may be one of support vector machine SVM, linear ...
Embodiment 2
[0037] The support vector machine SVM and the built-in kernel function of the kernel function are used as the linear kernel function linear for further explanation.
[0038] A debris flow early warning method based on the coupling of mechanism and machine learning, including the following steps:
[0039] (1) Construct a database of geological hazards and rainfall.
[0040] ① Debris flow disaster data: Collect the debris flow disaster data in the study area over the years. The disaster data includes the location (latitude and longitude) of the debris flow and the time when the debris flow occurred.
[0041] ② Underlying surface data: The small watershed units in the study area were extracted using the method of the paper "A Regional-Scale Method of Forecasting DebrisFlow Events Based on Water-Soil Coupling Mechanism". Number each small watershed unit (the maximum number is denoted as Nmax). The polygon file is then converted to a raster file with the same cell size as the dig...
PUM
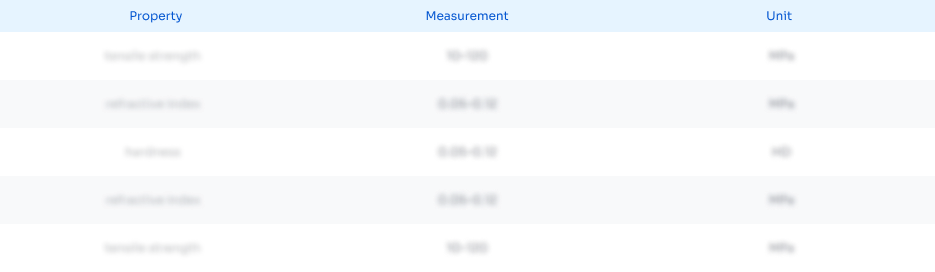
Abstract
Description
Claims
Application Information
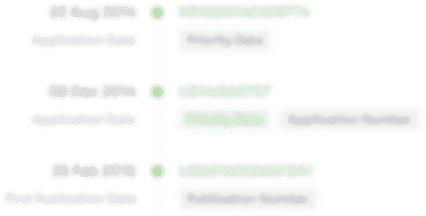
- R&D Engineer
- R&D Manager
- IP Professional
- Industry Leading Data Capabilities
- Powerful AI technology
- Patent DNA Extraction
Browse by: Latest US Patents, China's latest patents, Technical Efficacy Thesaurus, Application Domain, Technology Topic, Popular Technical Reports.
© 2024 PatSnap. All rights reserved.Legal|Privacy policy|Modern Slavery Act Transparency Statement|Sitemap|About US| Contact US: help@patsnap.com